Seeing Through Obstructions with Diffractive Cloaking
- Zheng Shi
- Yuval Bahat
- Seung-Hwan Baek
- Qiang Fu
- Hadi Amata
-
Xiao Li
- Praneeth Chakravarthula
- Wolfgang Heidrich
- Felix Heide
SIGGRAPH 2022
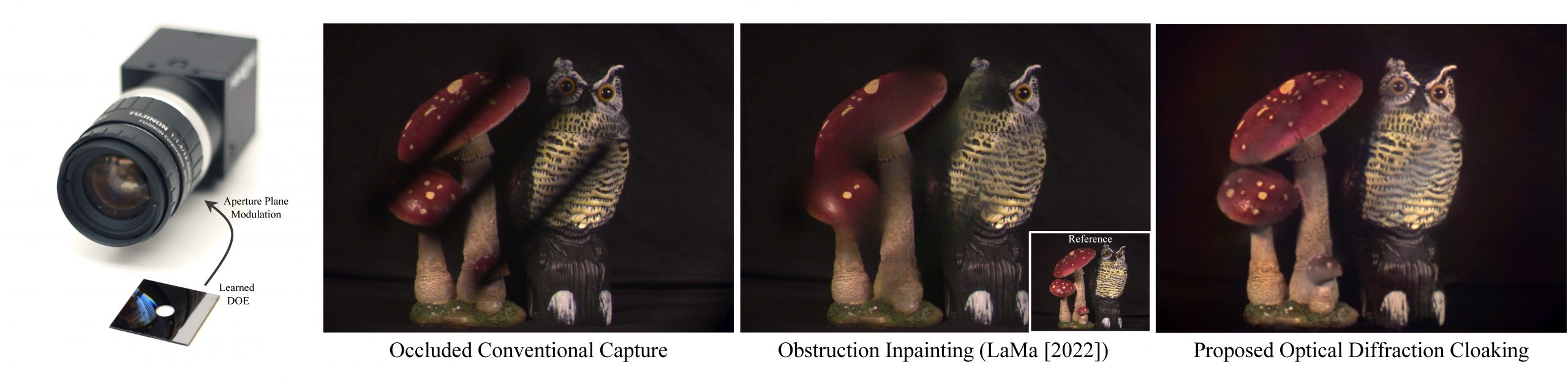
We propose a computational monocular camera that optically cloaks unwanted obstructions near the camera. Instead of inpainting the obstructed information post-capture, we learn a custom diffractive optical element at the aperture plane that acts as a depth-dependent scatterer. In conjunction with the optical element, we jointly optimize a feature-based deep learning reconstruction network to recover the unobstructed image.
Unwanted camera obstruction can severely degrade captured images, including both scene occluders near the camera and partial occlusions of the camera cover glass. Such occlusions can cause catastrophic failures for various scene understanding tasks such as semantic segmentation, object detection, and depth estimation. To overcome this, some use camera arrays that capture multiple redundant views of a scene to see around thin occlusions. Such multi-camera systems effectively form a large synthetic aperture, which can suppress nearby occluders with a large defocus blur, but significantly increase the overall form factor of the imaging setup.
In this work, we propose a monocular single-shot imaging approach that optically cloaks obstructions. Instead of relying on different camera views, we learn a diffractive optical element (DOE) that performs depth-dependent optical encoding, scattering nearby occlusions while allowing paraxial wavefronts emanating from background objects to be focused. We computationally reconstruct unobstructed images from these superposed measurements with a neural network that is trained jointly with the optical element of the proposed imaging system. We assess the proposed method in simulation and with an experimental prototype, validating that the proposed computational camera is capable of recovering occluded scene information in the presence of severe camera obstructions.
Diffractive Clocking in Action
Seeing Through Obstructions with Diffractive Cloaking
Zheng Shi, Yuval Bahat, Seung-Hwan Baek, Qiang Fu, Hadi Amata, Xiao Li,
Praneeth Chakravarthula, Wolfgang Heidrich, and Felix Heide
SIGGRAPH 2022
Learning Obstruction-aware Diffractive Optical Elements
We learn a DOE placed in front of a camera lens jointly with an optics-aware reconstruction network, to enable seeing through thin occluders close to the camera. To this end, we introduce a differentiable obstruction-aware image formation model that simulates the sensor capture of the proposed optical system.
The proposed camera (top left) modulates the latent scene at a long distance and the obstruction close to the observer (e.g., dirt on the wind shield) with the depth-dependent point-spread function of the learned optical element. The resulting sensor capture (top right) is fed into a feature deconvolution network (bottom) along with the depth-dependent point spread function (center). The entire computational imaging system, including optical and computational phases, is jointly learned (bottom left), resulting in a DOE that scatters light pertaining to nearby objects using a ring-shaped PSF, while preserving angular resolution for objects at optical infinity.
Learned Depth-dependent PSFs
We report the near-scene and far-scene PSFs and MTFs corresponding to the proposed learned optical design, and to other heuristic baseline optical designs that do not increase the aperture.
The proposed design achieves high MTF values at long distances corresponding to background scene depths, and disperses the radiance from nearby objects over the entire sensor. Specifically, the PSF at optical infinity resembles that of a conventional lens with a small focal spot, while the PSF for nearby distances resembles a large ring, with a radius prescribed by the maximum diffraction angle supported by the phase plate. Note that this PSF is obtained using our end-to-end learning approach, without using any design heuristics.
Experimental Prototype System
We implement the proposed method experimentally with the prototype system shown below. To this end, we fabricate the DOE designed for the front-facing automotive imaging task in a 16-level photolithography process on a fused silica wafer. To place the DOE on the aperture plane, instead of cutting an existing lens barrel, we relay the phase DOE to the aperture plane using a 4F system.
We measure the PSF of the entire optical system with (top row) and without (bottom row) the DOE. The measurements validate that the designed phase plate produces a ring-shaped PSF for close occluders, while resulting in a small spot size for far scene content. Manufacturing inaccuracies result in a 0th-order component (top left) at close distances, for which we compensate by providing the measured PSF to the reconstruction network.
Experimental Results
The proposed approach achieves a 1-5 dB PSNR gain over existing approaches when reconstructing occluded regions, by optically cloaking the obstruction while relying on the complementary reconstruction network to remove image aberrations, thus effectively allowing the occluded background to be “seen”.
The experimental captures below further validate that the proposed method is able to remove diverse obstructions and recover the hidden details, while existing image inpainting methods can merely attempt to “hallucinate” the missing content.
Each scene is first captured without the occluder to serve as a reference. We then introduce occlusions (here in the form of water drops) into the scene, and capture the scene using a conventional camera, which is later inpainted by a state-of-art method to serve as a baseline. Finally, we capture the scene with our proposed system, and reconstruct the image using our reconstruction network, to obtain the obstruction-free background scene.
Notice the details recovered by our method on the eagle’s head, flowers and stones.
Here we show the recovery for the case of splashes of soil on the camera coverglass.
Notice that the proposed method is able to recover text details such as the number 50 on the book cover, unlike the alternatives.
In this example we use black tapes to simulate fence-like thin occluders.
Our method is able to recover small branches of the coral, as well as the pose of the figurine, while the inpainting method blends the objects into the black background, and even hallucinates a face on the head of the figurine, which in reality does not have any surface details.
Related Publications
[1]Ethan Tseng, Shane Colburn, James Whitehead, Luocheng Huang, Seung-Hwan Baek, Arka Majumdar, Felix Heide. Neural Nano-Optics for High-quality Thin Lens Imaging. Nature Communications 2021
[2] Seung-Hwan Baek and Felix Heide. Polka Lines: Learning Structured Illumination and Reconstruction for Active Stereo. In IEEE International Conference on Computer Vision and Pattern Recognition (CVPR), 2021
[3] Ethan Tseng, Ali Mosleh, Fahim Mannan, Karl St-Arnaud, Avinash Sharma, Yifan Peng, Alexander Braun, Derek Nowrouzezahrai, Jean-François Lalonde, and Felix Heide. Differentiable Compound Optics and Processing Pipeline Optimization for End-to-end Camera Design. ACM Transactions on Graphics (TOG), 40(2):18, 2021
[4] Ilya Chugunov, Seung-Hwan Baek, Qiang Fu, Wolfgang Heidrich, and Felix Heide. Mask-ToF: Learning Microlens Masks for Flying Pixel Correction in Time-of-Flight Imaging. In IEEE International Conference on Computer Vision and Pattern Recognition (CVPR), 2021