Polka Lines: Learning Structured Illumination and Reconstruction for Active Stereo
CVPR 2021 (Oral)
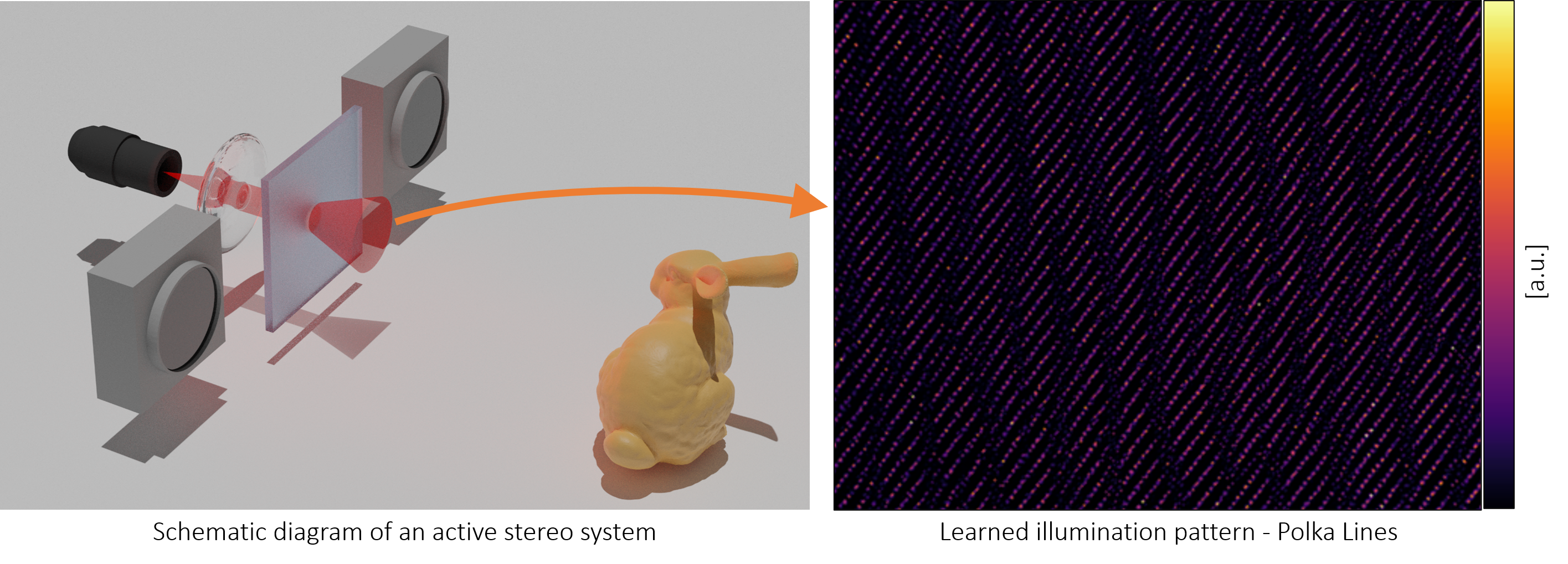
Active stereo cameras that recover depth from structured light captures have become a cornerstone sensor modality for 3D scene reconstruction and understanding tasks across application domains. Active stereo cameras project a pseudo-random dot pattern on object surfaces to extract disparity independently of object texture. Such hand-crafted patterns are designed in isolation from the scene statistics, ambient illumination conditions, and the reconstruction method. In this work, we propose a method to jointly learn structured illumination and reconstruction, parameterized by a diffractive optical element and a neural network, in an end-to-end fashion. To this end, we introduce a differentiable image formation model for active stereo, relying on both wave and geometric optics, and a trinocular reconstruction network. The jointly optimized pattern, which we dub “Polka Lines,” together with the reconstruction network, makes accurate active-stereo depth estimates across imaging conditions. We validate the proposed method in simulation and using with an experimental prototype, and we demonstrate several variants of the Polka Lines patterns specialized to the illumination conditions.
Paper
Seung-Hwan Baek, Felix Heide
Polka Lines: Learning Structured Illumination and Reconstruction for Active Stereo
CVPR 2021
Pipeline Design of Conventional Active Stereo Systems
Active stereo cameras equip a stereo camera pair with an illumination module that projects a fixed pattern onto a scene so that, independently of surface texture, stereo correspondence can be reliably estimated. As such, active stereo methods allow for single-shot depth estimates at high resolutions using low-cost diffractive laser dot modules and conventional CMOS sensors. However, they struggle with extreme ambient illumination and complex scenes, prohibiting reliable depth estimates in uncontrolled in-the-wild scenarios. These limitations are direct consequences of the pipeline design of existing active stereo systems, which handengineer the illumination patterns and the reconstruction algorithms in isolation.
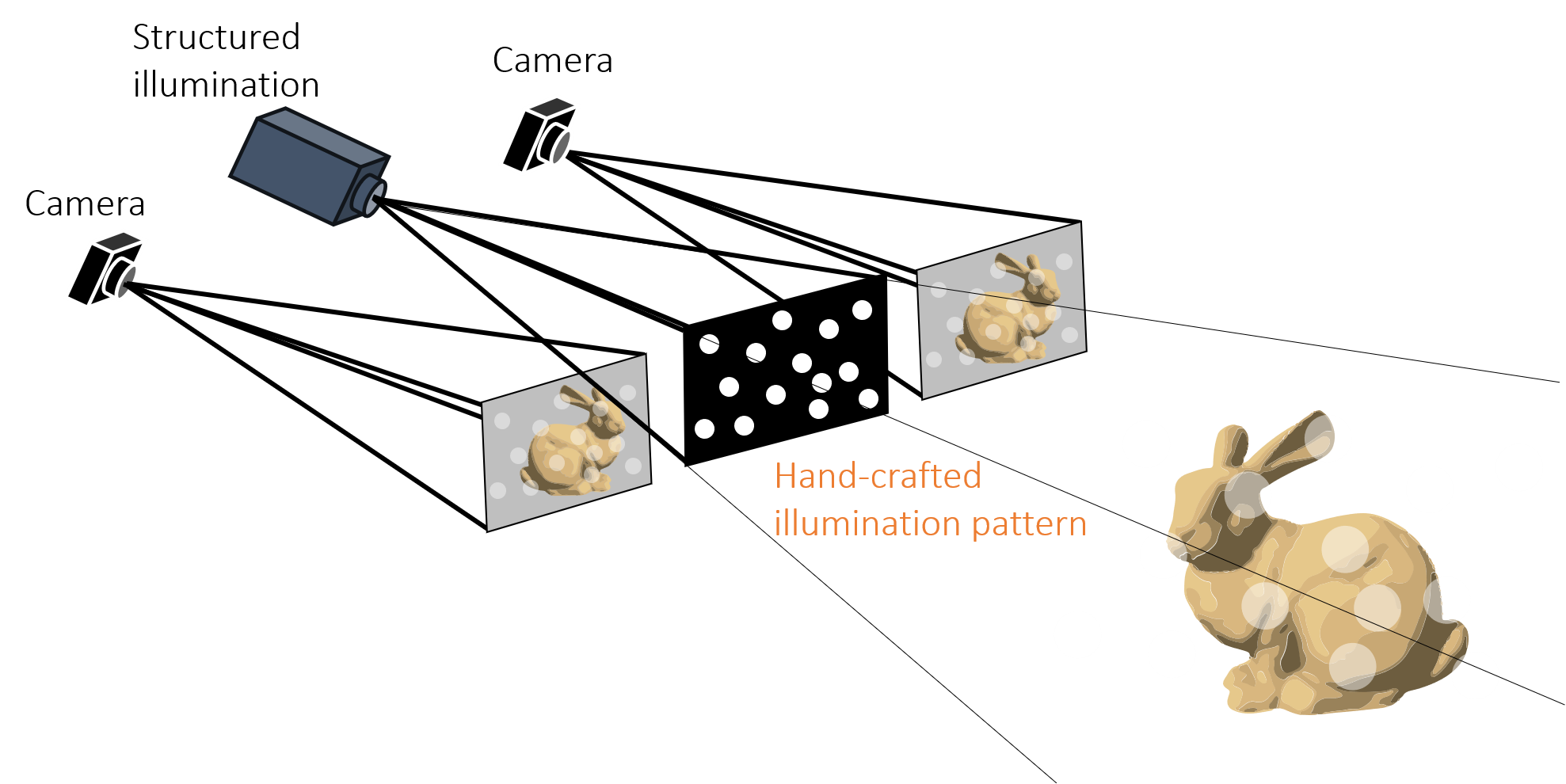
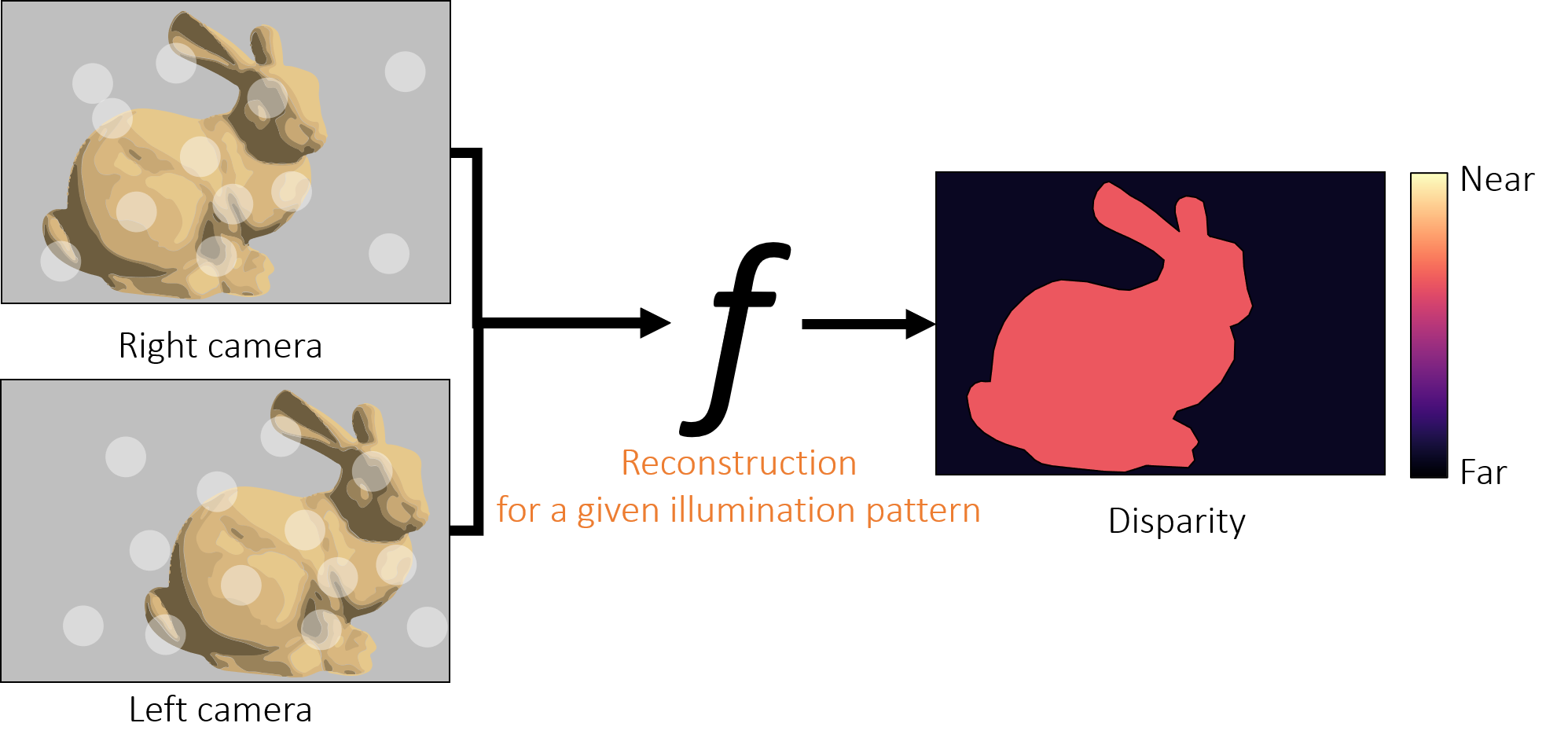
Joint End-to-end Design for Active Stereo
In this work, we propose an active-stereo design method that jointly learns illumination patterns and a reconstruction algorithm, parameterized by a DOE and a neural network, in an end-to-end manner.
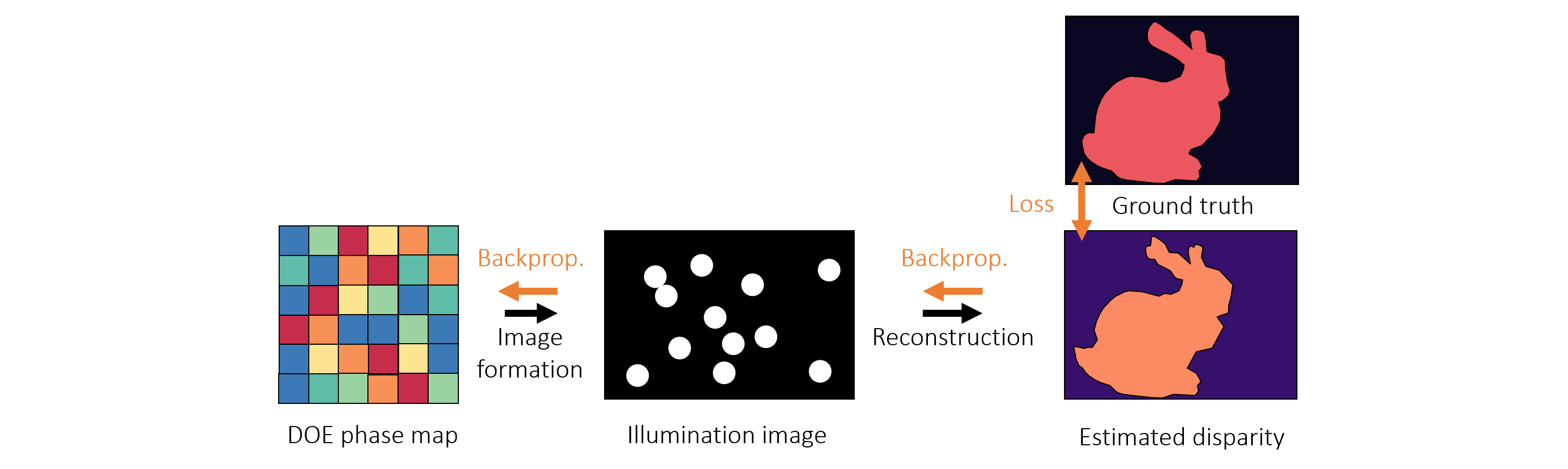
Differentiable Image Formation and Reconstruction
To enable the end-to-end design, we propose a differentiable imaging and reconstruction pipeline that can be used to jointly optimize the entire stereo camera pipeline, from illumination to reconstruction. We simulate the entire image formation process starting from the illumination module and ending with capture by the stereo cameras. Our hybrid image formation model exploits both geometric and wave optics in order to efficiently perform this forward simulation in a differentiable manner.
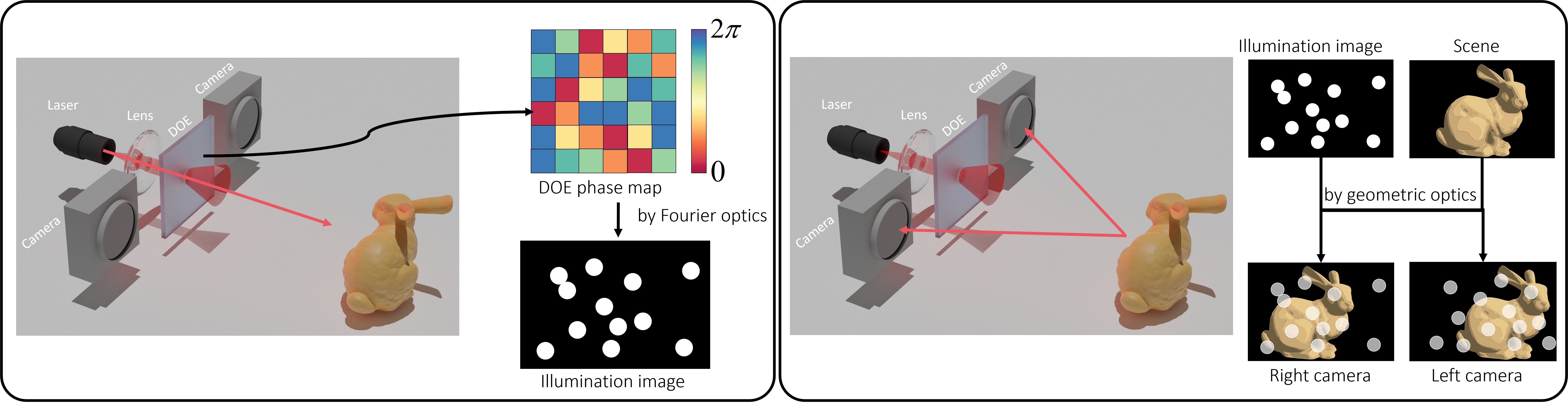
From the simulated stereo images, we reconstruct a depth map using a trinocular neural network exploiting the known illumination pattern being optimized. Reconstruction loss is computed between the estimated and ground-truth depth, and this loss is backpropagated to the illumination module and the network.
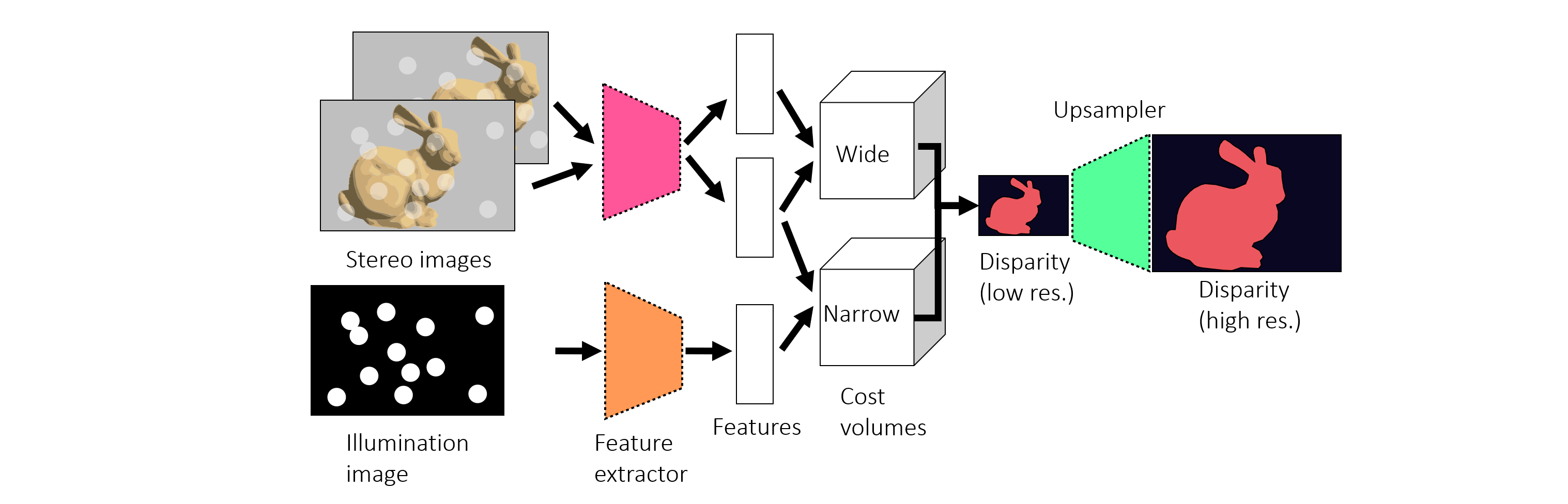
Learned Illumination - Polka Lines
The proposed Polka Lines design is the result of the proposed optimization method. We can interpret the performance of this pattern by analyzing the structure of the Polka Lines patterns. First, each dot in a line of dots has varying intensity levels, in contrast to the constant-intensity heuristic patterns. Second, the orientations of Polka Lines are locally varying, which is a discriminative feature for correspondence matching. Our end-to-end method enables designing scene-specific illumination patterns. We show examples of learned illumination patterns that are adapted for different noise levels and ambient light intensities. These computationally learned patterns provide insight into effective pattern design for specific target environments.
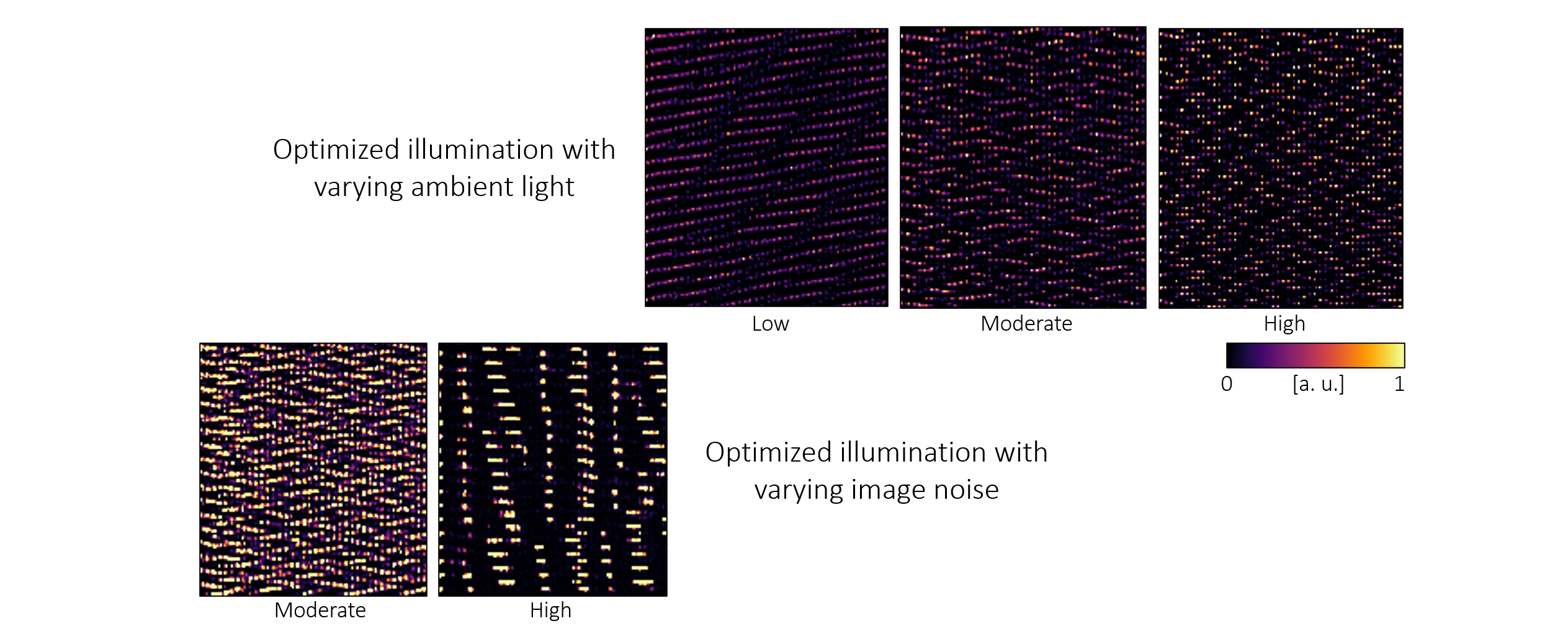
Experimental Prototype
We built an experimental prototype with three learned DOEs mounted on a rotation stage and stereo IR cameras mounted side-by-side. The rotation stage allows for switchable illumination using each DOE. The learned Polka Lines are projected onto a scene by passing a collimated light wave through the phase-modulating DOEs.
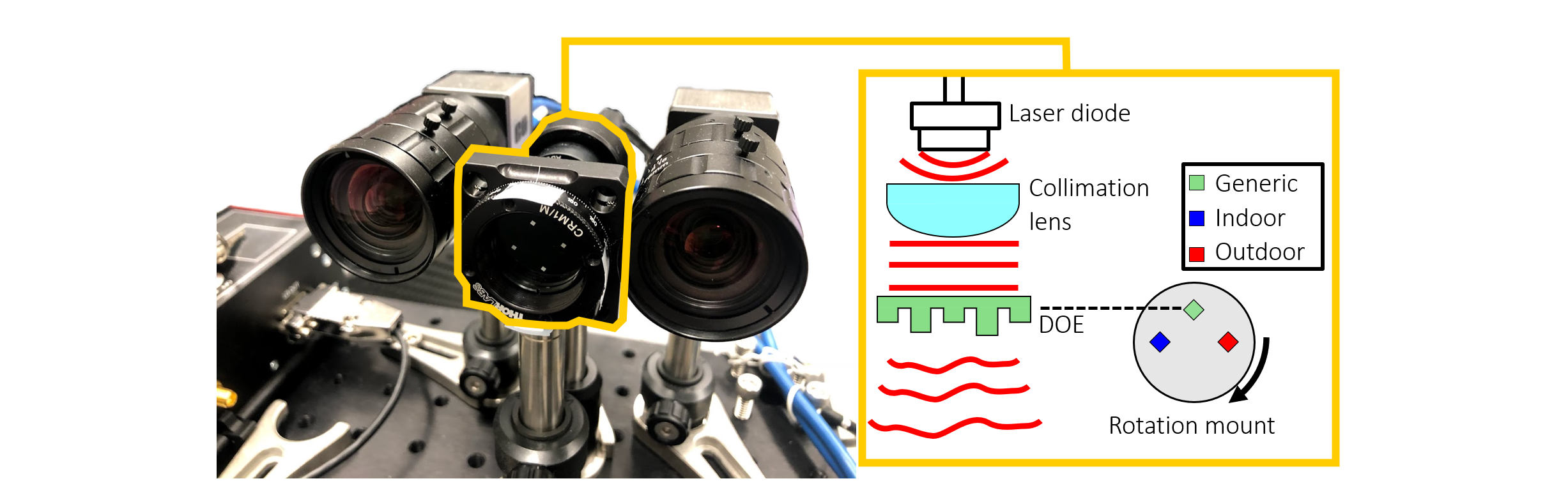
Real-world Results
Our system acquires accurate disparity for challenging scenes. We show here examples containing complex objects
including textureless surfaces under diverse environments from indoor illumination to outdoor sunlight. In addition, we developed a live-capture system that acquires stereo images and estimates a disparity map at 10 frames per second (FPS). Target objects are a low-reflectance diffuse bag, a highly specular red stop sign, a ColorChecker, and a transparent bubble wrap.
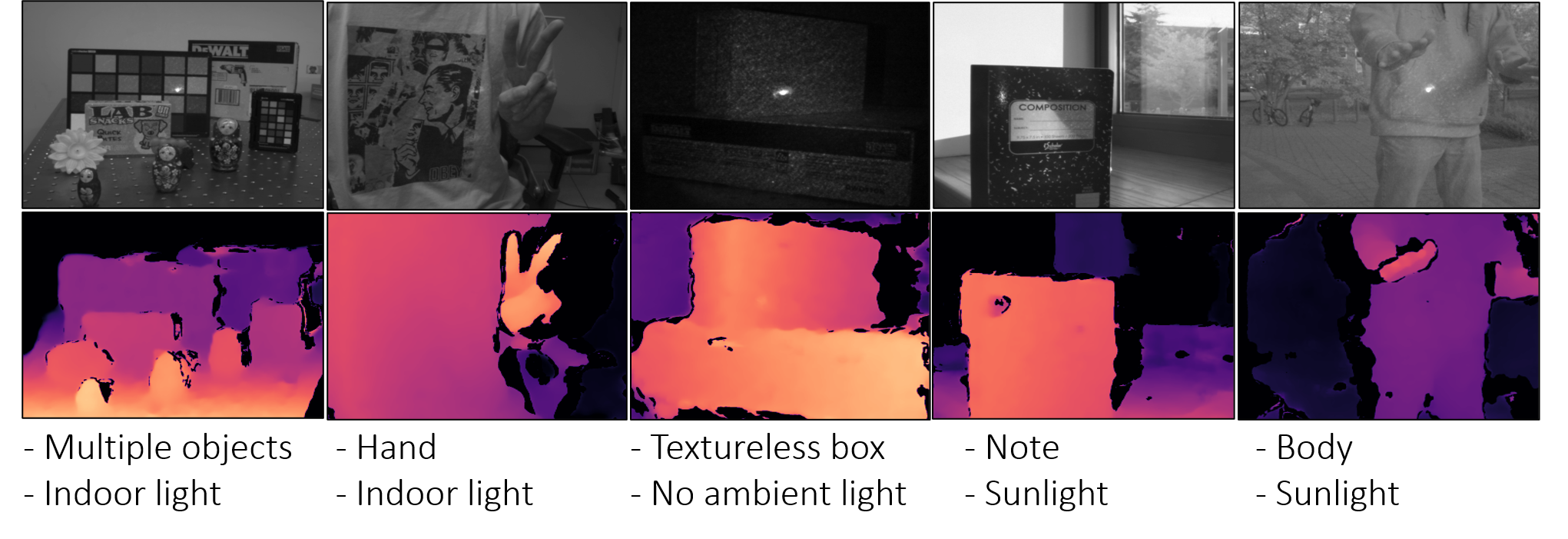