Neural Nano-Optics for High-quality Thin Lens Imaging
Nature Communications

We present neural nano-optics, offering a path to ultra-small imagers, by jointly learning a metasurface optical layer and neural feature-based image reconstruction. Compared to existing state-of-the-art hand-engineered approaches, neural nano-optics produce high-quality wide-FOV reconstructions corrected for chromatic aberrations.
Nano-optic imagers that modulate light at sub-wavelength scales could unlock unprecedented applications in diverse domains ranging from robotics to medicine. Although metalenses offer a path to such ultra-small imagers, existing method have achieved image quality far worse than bulky refractive alternatives, fundamentally limited by aberrations at large apertures and low f-numbers. In this work, we close this performance gap by presenting the first neural nano-optics. We devise a fully differentiable learning method that learns a metasurface physical structure in conjunction with a novel, neural feature-based image reconstruction algorithm. Experimentally validating the proposed method, we achieve an order of magnitude lower reconstruction error. As such, we present the first high-quality, nano-optic imager that combines the widest field of view for full-color metasurface operation while simultaneously achieving the largest demonstrated 0.5 mm, f/2 aperture.
Paper
Ethan Tseng, Shane Colburn, James Whitehead, Luocheng Huang, Seung-Hwan Baek, Arka Majumdar, Felix Heide
Neural Nano-Optics for High-quality Thin Lens Imaging
Nature Communications
Neural Nano-Optics in the news
Neural Nano-Optics was voted by Optica as one of the top 30 breakthrough ideas in optics in 2021 and has been covered by numerous news and media outlets around the world.
Video
Video Summary of Neural Nano-Optics. We propose a computational imaging method for end-to-end learning of ultra-thin meta-surface lenses and the reconstruction of captured measurements. Although the size of a rice grain, neural nano-optics almost achieve the same quality as bulky compound lenses.
Salt Grain-sized Cameras
The ultracompact camera we propose uses metasurface optics at the size of a coarse salt grain and can produce crisp, full-color images on par with a conventional compound camera lens 500,000 times larger in volume.
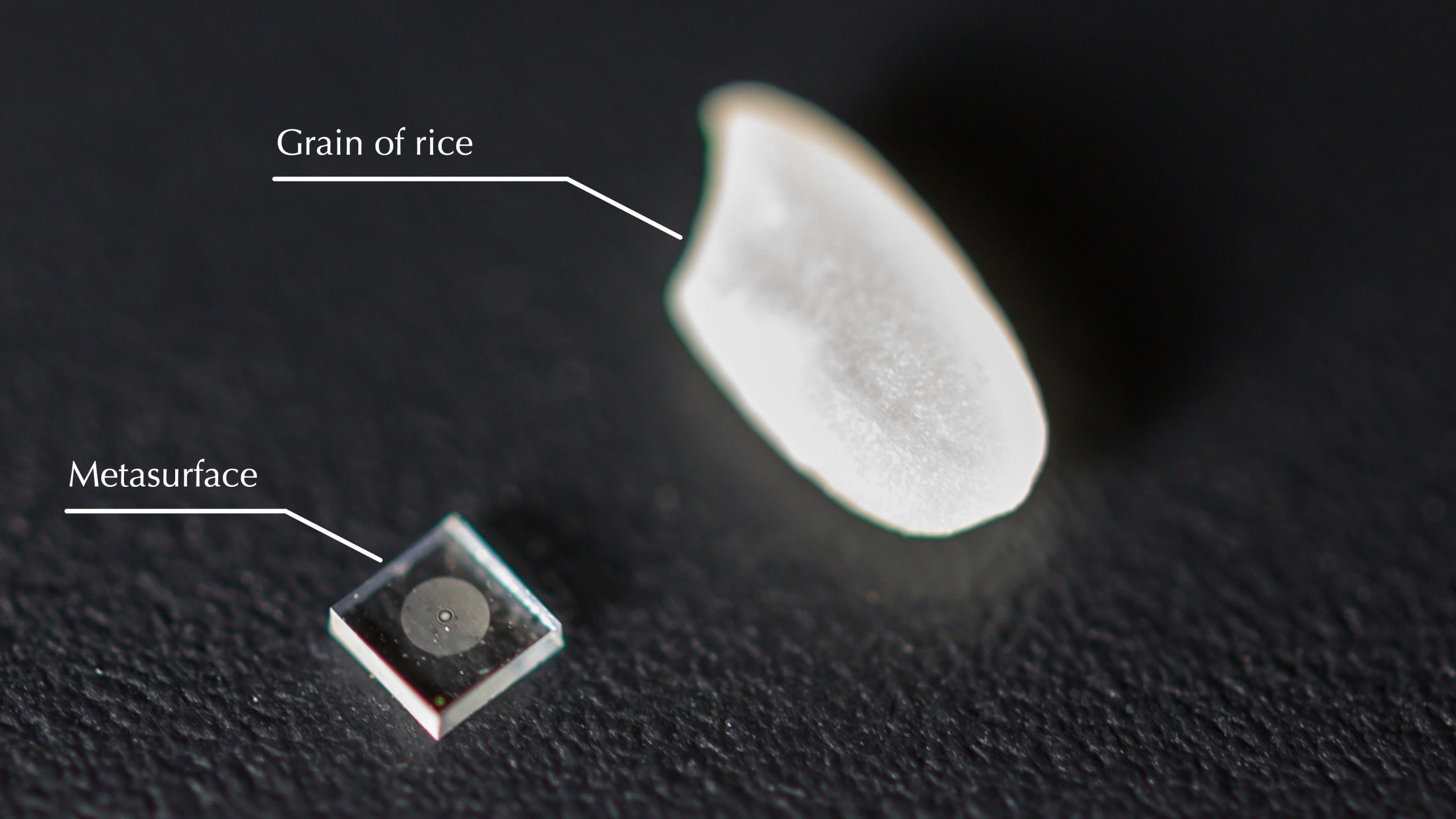
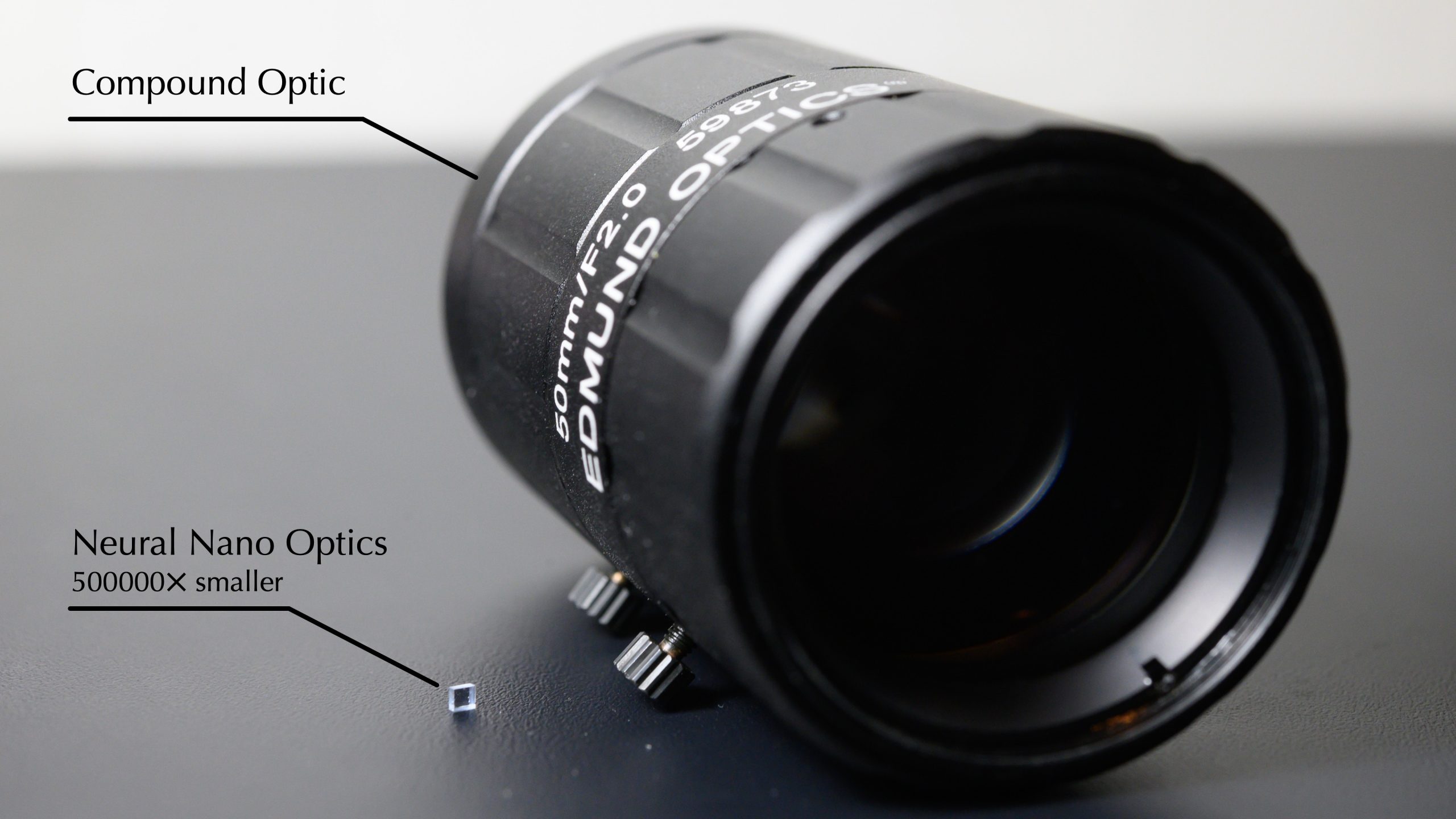
Comparisons against State-of-the-art
Neural nano-optics outperform existing state-of-the-art metasurface lens designs in broadband and it is the first meta-optic imager that achieves high-quality, wide field-of-view color imaging.
Comparisons against Conventional Cameras
Neural nano-optics can recover images with quality comparable to conventional cameras with bulky refractive compound lenses.
Metasurface Proxy Model
We learn a nano-optic by using an efficient differentiable proxy function that maps phase values to spatially varying PSFs. This allows our approach to be more than three orders of magnitude more efficient than full-wave simulation methods such as FTDT.
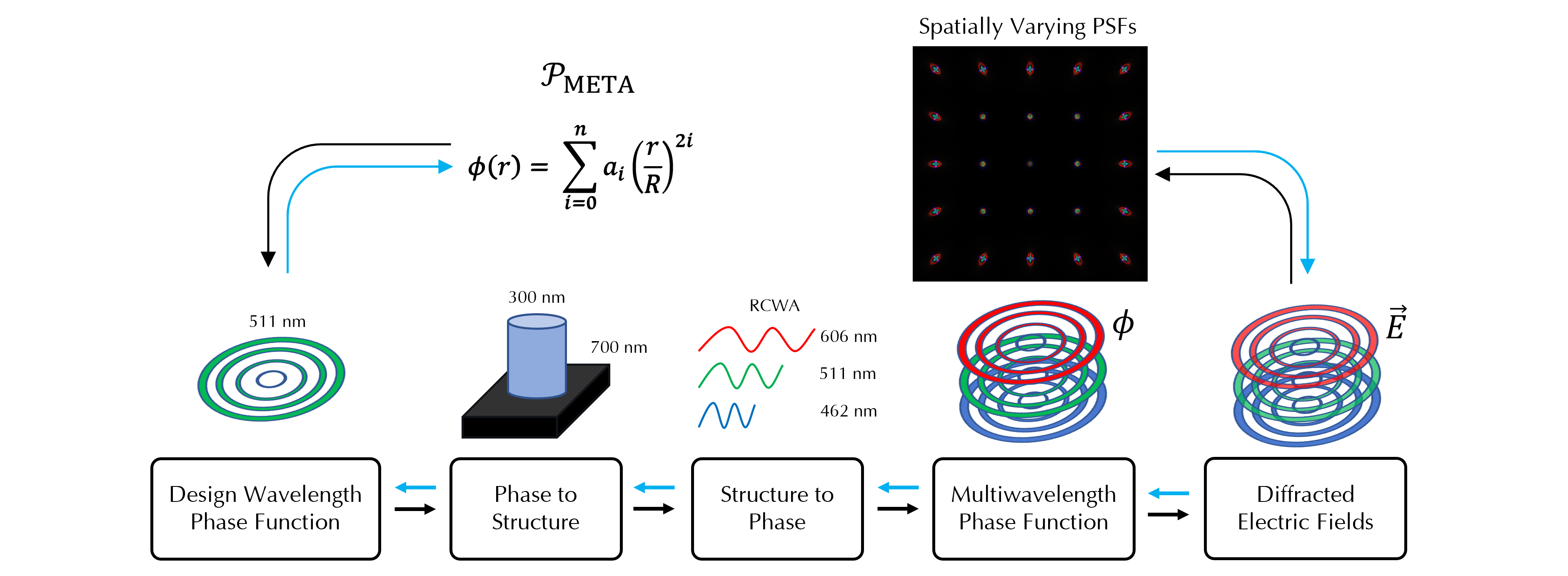
Feature-based Deconvolution
To recover images from the measured data, we propose a feature-based deconvolution method that employs a differentiable inverse filter together with neural networks for feature extraction and refinement. This combination allows us to learn effective features that facilitate accurate and physics-based deconvolution utilizing the knowledge of the PSF to promote generalizability.
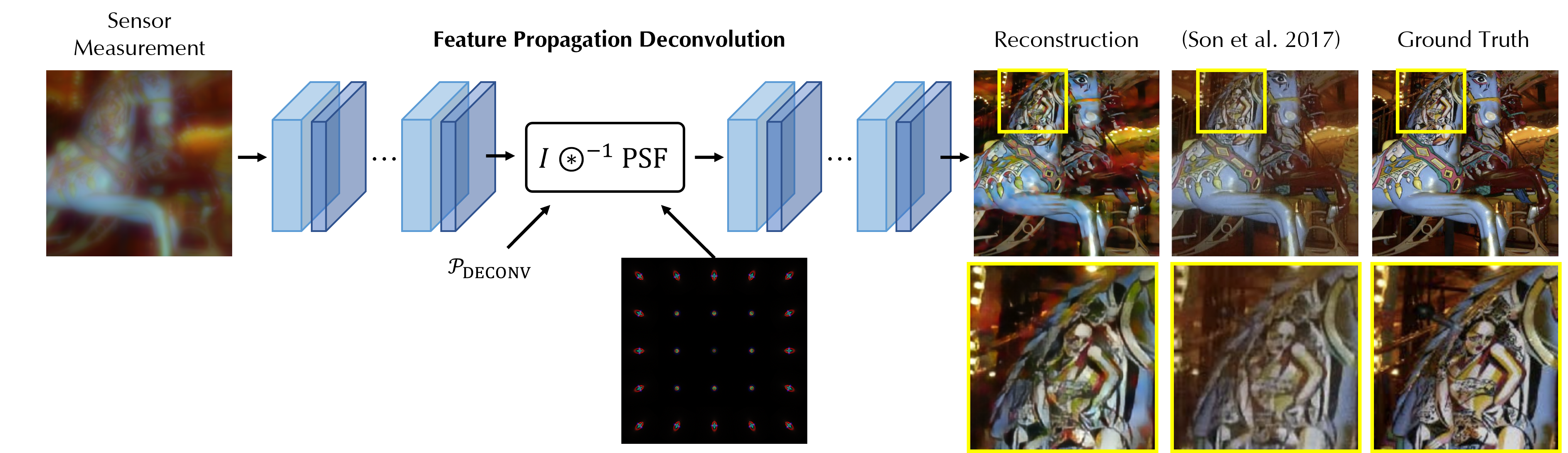
Learning Meta-Optics in an End-to-end Fashion
With the metasurface proxy and neural deconvolution model in hand, comprising a fully differentiable imaging pipeline, we design the proposed nano-camera in an end-to-end fashion. The figure below illustrates the learning method and a corresponding optimization run.
Diffractive Lens Achromatic Capacity (DLAC)
Our optimized meta-optic design meets several criteria that were not met by previous methods. The nano-camera allows us to capture full-color images over a wide field-of-view. Furthermore, our aperture is the largest demonstrated for meta-optics at 500 microns, allowing for increased light collection. Our optic also exhibits a low f-number, allowing for the optic to be placed extremely close to the camera sensor. No previous metasurface has demonstrated imaging with this combination of large aperture, large field-of-view, small f-number, and large fractional bandwidth. Previously, achieving any one of these metrics came at the cost of some other, for example, one could achieve a low f-number by sacrificing the aperture size. To formally quantify our design specs, we propose a new metric called the Diffractive Lens Achromatic Capacity.
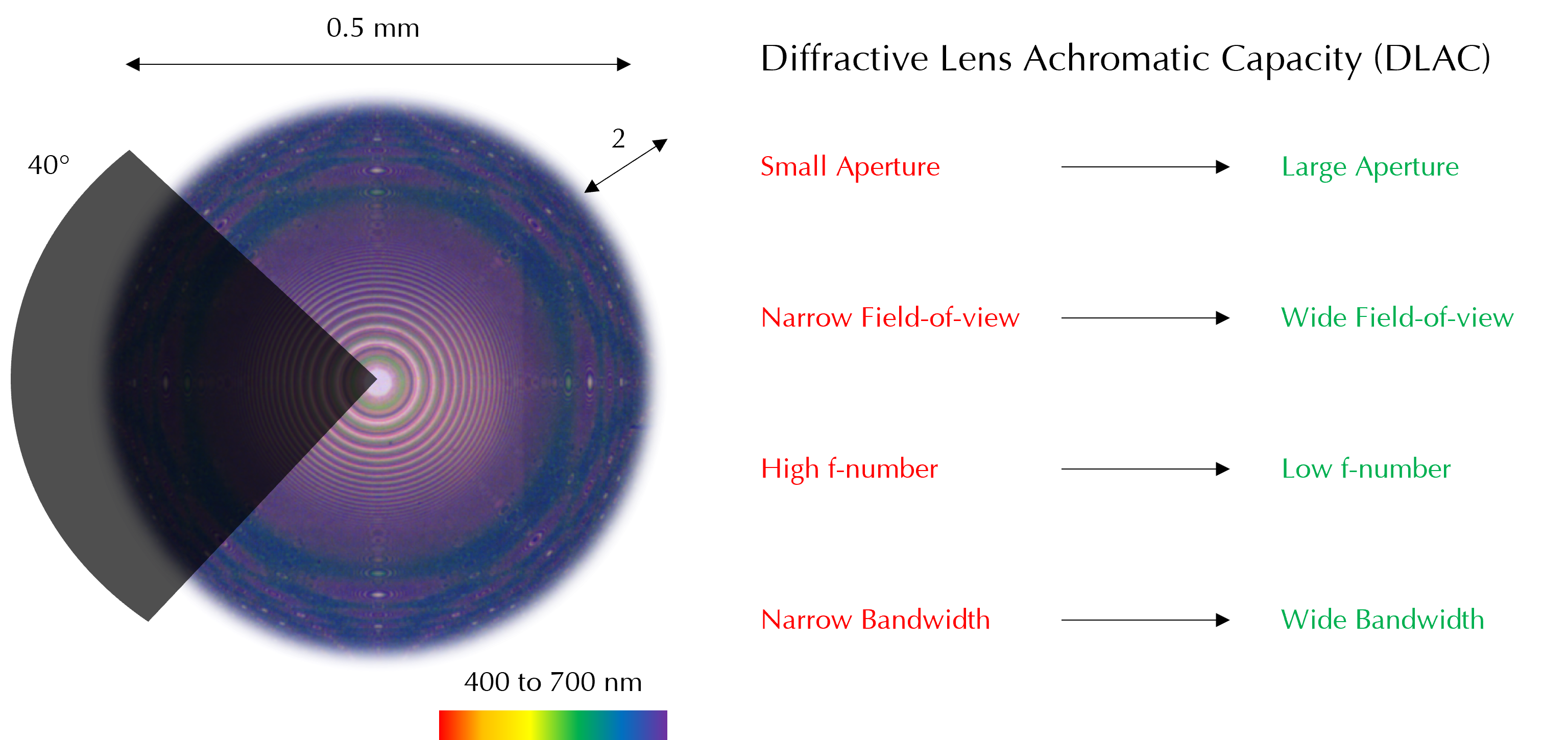
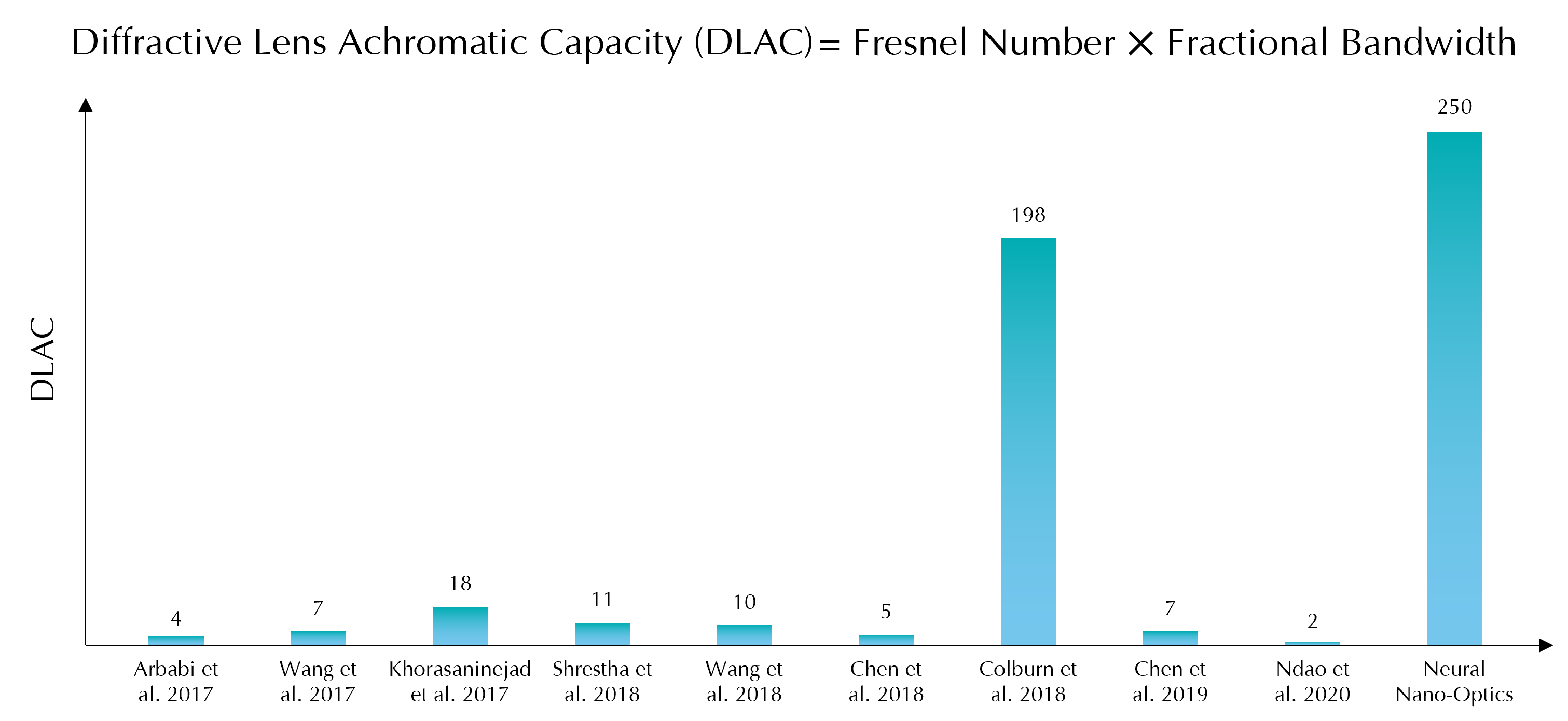
Meta-optic PSFs
We visualize the simulated PSFs for each design. Our optimization produces a meta-optic whose spatial PSFs are compact across all wavelengths and are slowly varying across field angles. Other designs exhibit PSFs with greater blur, especially for the red wavelength, and with rapid variation across field angles. The PSF corresponding to the cubic design contains large tails that cause the overall size of the PSF to be much larger than that of all other designs. As such, we only display the center crop of cubic design PSFs from Colburn et al.
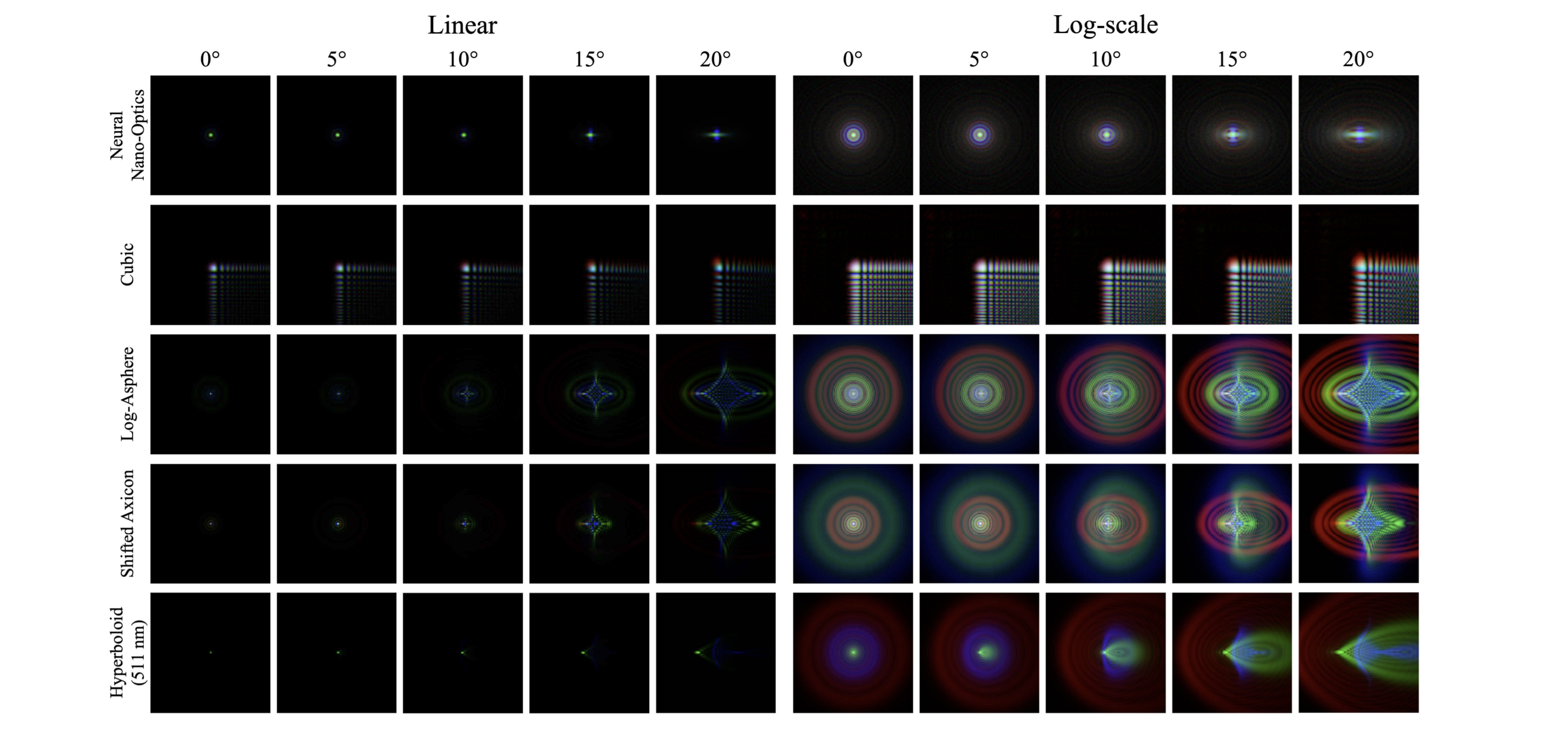
Siemens Resolution Chart
Siemens Resolution Star captures compared to heuristic designs by Colburn et al. and a refractive compound lens. Computational reconstruction with our neural nano-optic imager recovers spatial resolution in broadband almost matching the performance of a refractive compound lens. See Supplemental Material for USAF 1951 chart comparisons.
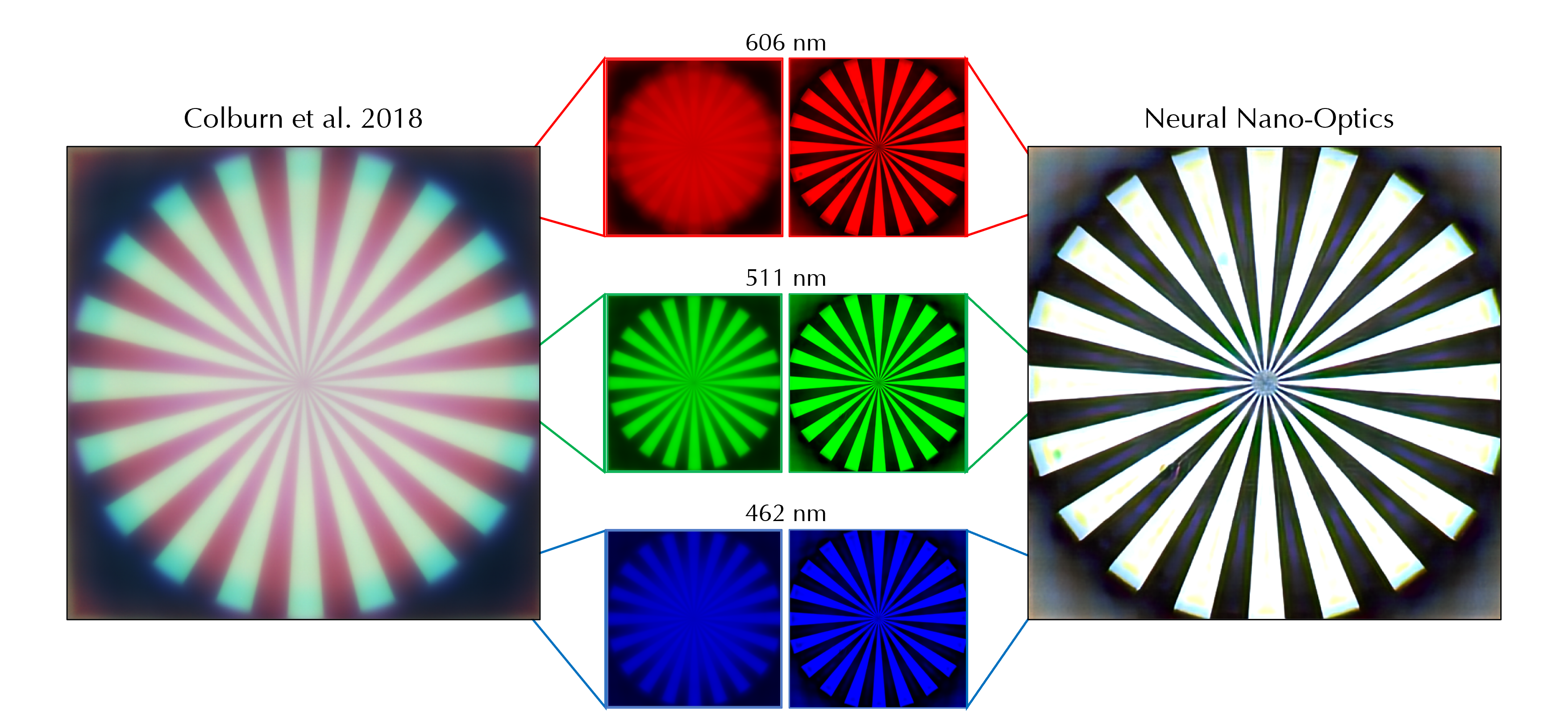
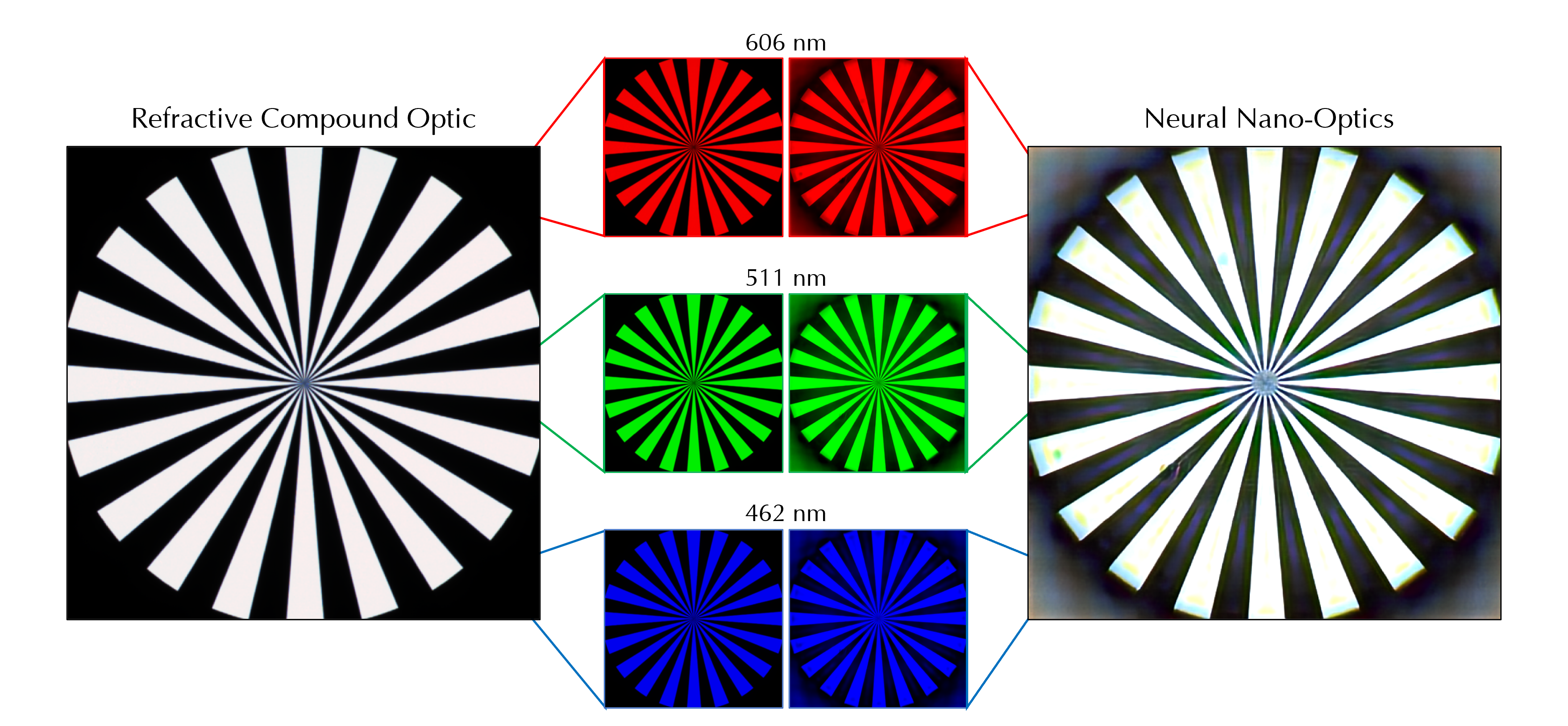
Related Publications
[1] Ethan Tseng, Ali Mosleh, Fahim Mannan, Karl St-Arnaud, Avinash Sharma, Yifan Peng, Alexander Braun, Derek Nowrouzezahrai, Jean-François Lalonde, and Felix Heide. Differentiable Compound Optics and Processing Pipeline Optimization for End-to-end Camera Design. ACM Transactions on Graphics (TOG), 40(2):18, 2021
[2] Seung-Hwan Baek and Felix Heide. Polka Lines: Learning Structured Illumination and Reconstruction for Active Stereo. In IEEE International Conference on Computer Vision and Pattern Recognition (CVPR), 2021
[3] Ilya Chugunov, Seung-Hwan Baek, Qiang Fu, Wolfgang Heidrich, and Felix Heide. Mask-ToF: Learning Microlens Masks for Flying Pixel Correction in Time-of-Flight Imaging. In IEEE International Conference on Computer Vision and Pattern Recognition (CVPR), 2021
[4] Qilin Sun, Ethan Tseng, Qiang Fu, Wolfgang Heidrich, and Felix Heide. Learning Rank-1 Diffractive Optics for Single-shot High Dynamic Range Imaging. In IEEE International Conference on Computer Vision and Pattern Recognition (CVPR), 2020