Spatially Varying Nanophotonic Neural Networks
The explosive growth in computation and energy cost of AI has spurred interest in alternative computing modalities to conventional electronic processors. Photonic processors, which use photons instead of electrons, promise optical neural networks with ultra-low latency and power consumption. However, existing optical neural networks, limited by their designs, have not achieved the recognition accuracy of modern electronic neural networks. We bridge this gap by embedding parallelized optical computation into flat camera optics that perform neural network computations during capture, before recording on the sensor. We instantiate this network inside the camera lens with a nanophotonic array with angle-dependent responses. Combined with a lightweight electronic backend of about 2K parameters, our reconfigurable nanophotonic neural network achieves 72.76% accuracy on CIFAR-10, surpassing AlexNet (72.64%), and advancing optical neural networks into the deep learning era. Since almost all computes are realized passively at the speed of light with near-zero energy consumption, we achieve more than 200-fold faster computation compared to existing GPU-based inference.
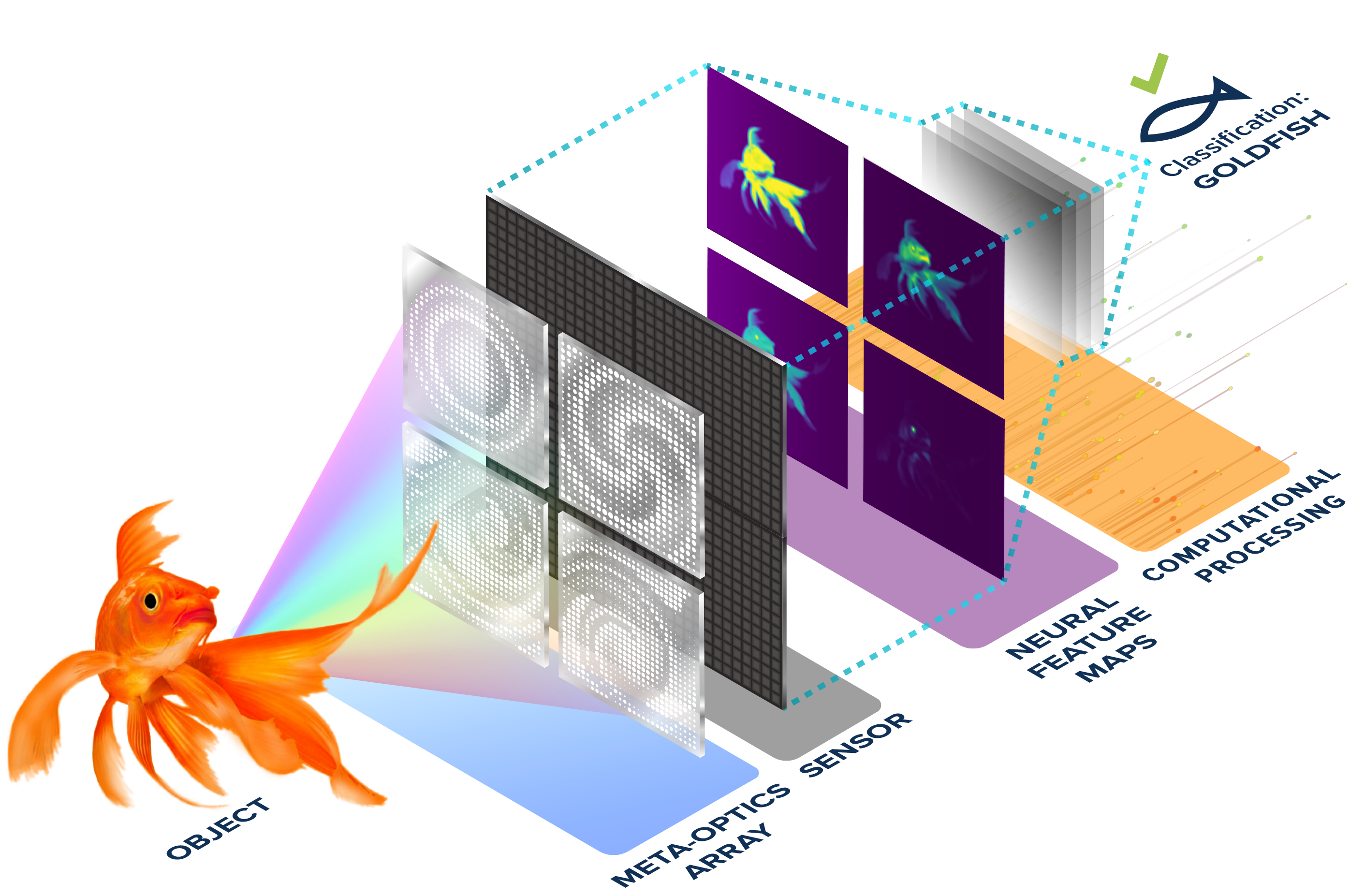
Kaixuan Wei, Xiao Li, Johannes Froech, Praneeth Chakravarthula, James Whitehead, Ethan Tseng, Arka Majumdar, Felix Heide
Spatially Varying Nanophotonic Neural Networks
Science Advances
Feature Extraction at the Speed of Light
Experimental prototype performing classification with a nanophotonic metasurface array mounted directly on the sensor cover glass. The proposed array performs feature extracting simultaneously with focusing at the speed of light.
We show here our prototype system in action—the optical feature extraction and image recognition on-the-fly. The proposed optical system is not only a photonic accelerator but also an ultra-compact computational camera that directly operates on the light from the environment before the analog to digital conversion.
Neural Optical Compute with Near-Zero Energy Consumption
Increasing demands for high-performance artificial intelligence in the last decade have levied immense pressure on computing architectures across domains. Although electronic microprocessors have undergone drastic evolution over the past 50 years, the end of voltage scaling has made power consumption the principal factor limiting further improvements in computing performance. Overcoming this limitation and radically reducing compute latency and power consumption could drive unprecedented applications from low-power edge computation in the camera. To this end, we investigate moving this computation to the optical side at near-zero energy cost, departing from conventional computer vision systems, which first focus an image onto a sensor and then run neural network inference on the sensor readout, all in the electronic hardware at high energy consumption.
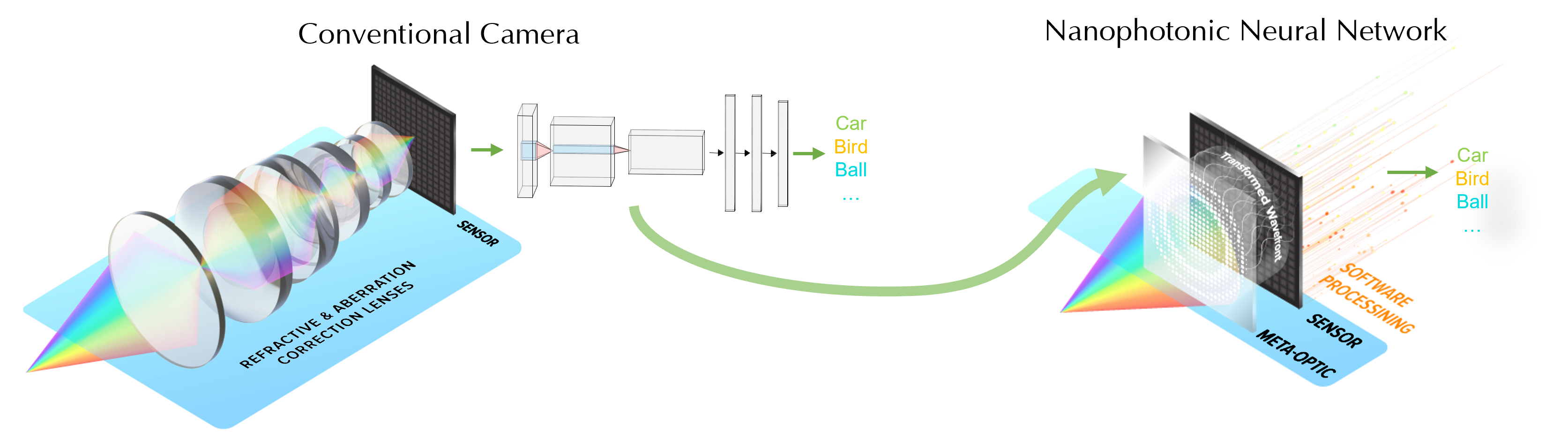
AI-designed Nanophotonic Vision System
(Row 1) The spatially varying nanophotonic neural network (SVN3) is an optoelectronic neuromorphic computer that comprises a metalens array nanophotonic front-end and a lightweight electronic back-end for high-level vision tasks (e.g., image classification or semantic segmentation). (Row 2) The metalens array front-end consists of 50 metalens elements that are inversely designed and optimized by AI techniques. This nanophotonic front-end has an ultra-small form factor comparable to a pencil eraser while performing parallel multichannel convolutions at the speed of light without any power consumption.
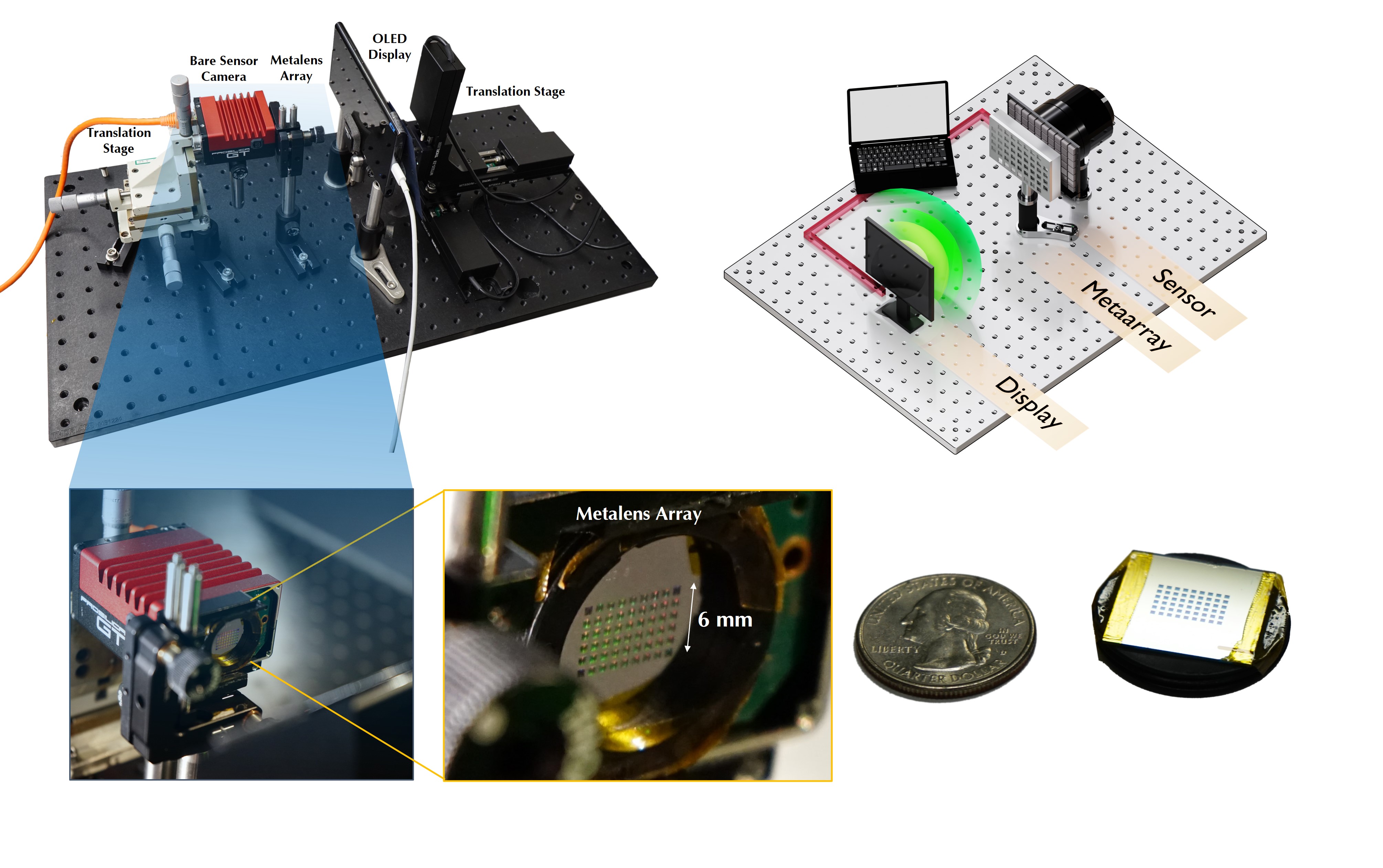
Large-Kernel Spatially-Varying Nanophotonic Neural Networks
(Left) We exploit the ability of a lens system to perform large-kernel spatially-varying convolutions tailored specifically for image recognition and semantic segmentation. These operations are performed during the capture before the sensor makes a measurement. (Right) We learn large kernels via low-dimensional reparameterization techniques (i.e., large-kernel factorization and low-rank spatially varying convolution) which circumvent spurious local extrema caused by direct optimization, leading to powerful kernels that can be deployed optically. The resulting optical feature maps are then fed into a minimalist designed electronic neural network for final prediction.
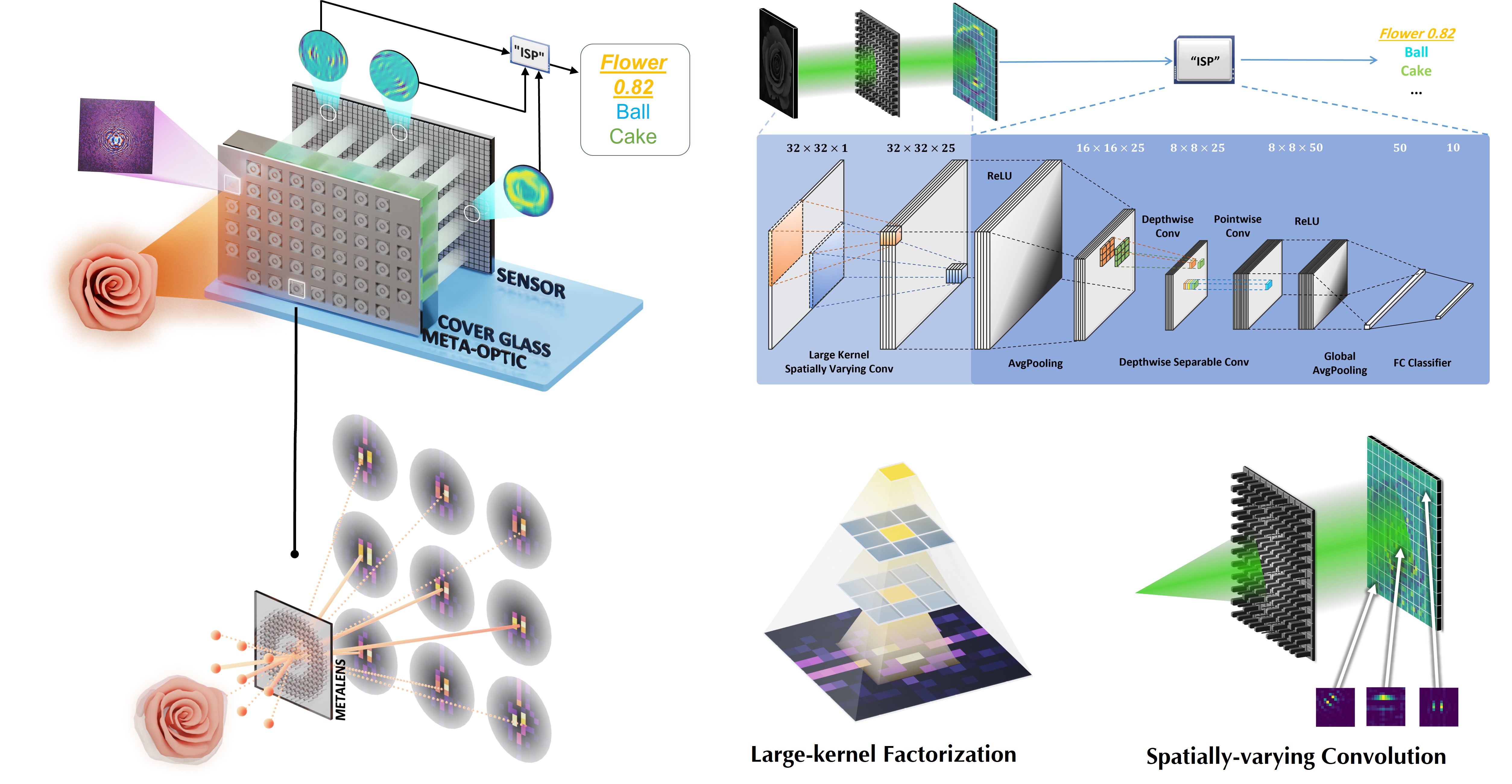
High-level Vision Inference with 99.88% Optical Compute
By on-chip integration of the flat-optics frontend (> 99% FLOPs) with an extremely lightweight electronic backend (< 1% FLOPs), we achieve higher classification performance than modern fully-electronic classifiers (AlexNet) while simultaneously reducing the number of electronic parameters by four orders of magnitude, thus bringing optical neural networks into the modern deep learning era.
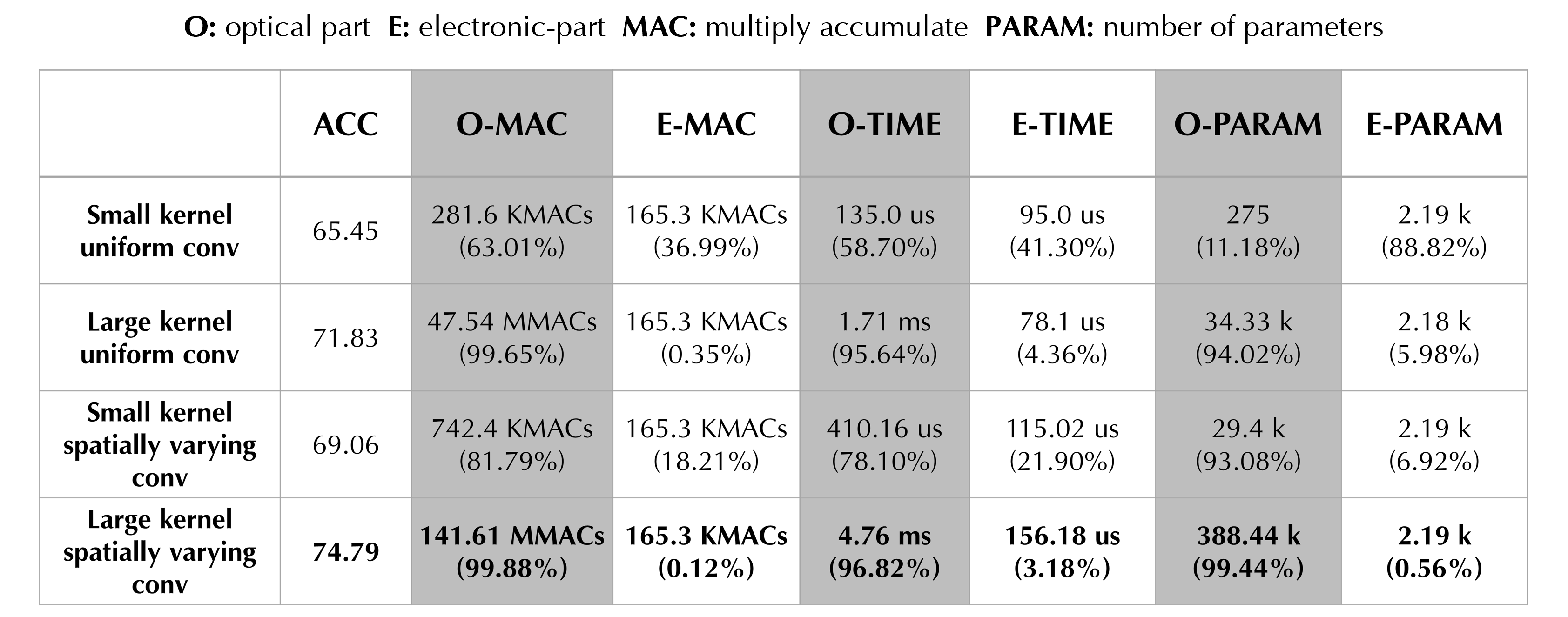
Note that the true optical runtime is a function of the optical path length and is ≈ 3 ps in our implementation. We report here the runtime numbers of the optical model component when implemented electronically for reference, i.e., higher is better.
Live Image Classification on Diverse Datasets
Additional live classification results of the system in action. The slow-motion movie showcases the accurate predictions of various object classes with the proposed experimental prototype.
(Left) Our approach achieves an image classification accuracy of (top-1) 72.76% on CIFAR-10 and (top-5) 48.64% on (1000-class) ImageNet, shrinking the gap between photonic and electronic artificial intelligence. (Right) It ensures generalization to diverse vision tasks [CIFAR-100, Flower-102, Food-101, Pet-37] without needing to fabricate new optics.
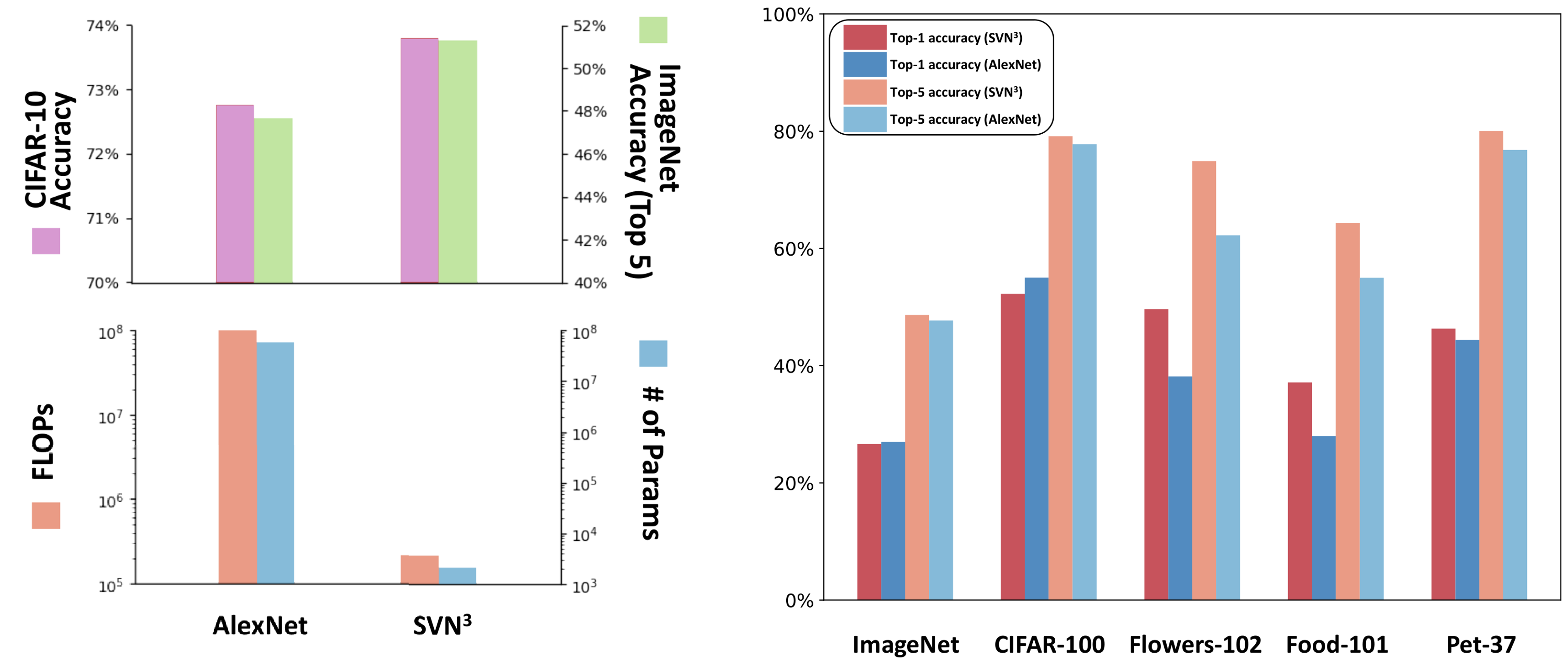
Related Publications
[1] Ethan Tseng, Shane Colburn, James Whitehead, Luocheng Huang, Seung-Hwan Baek, Arka Majumdar, Felix Heide. Neural Nano-Optics for High-quality Thin Lens Imaging. Nature Communications, 2021
[2] Hanyu Zheng, Quan Liu, You Zhou, Ivan I. Kravchenko, Yuankai Huo, Jason G. Valentine. Meta-optic Accelerators for Object Classifiers. Science Advance, 2022
[3] Hanyu Zheng, Quan Liu, Ivan I. Kravchenko, Xiaomeng Zhang, Yuankai Huo, Jason G. Valentine. Multichannel Meta-imagers for Accelerating Machine Vision. Nature Nanotechnology, 2024