Neural Exposure Fusion for High-Dynamic Range Object Detection
-
Emmanuel Onzon
-
Maximilian Bömer
- Fahim Mannan
- Felix Heide
CVPR 2024
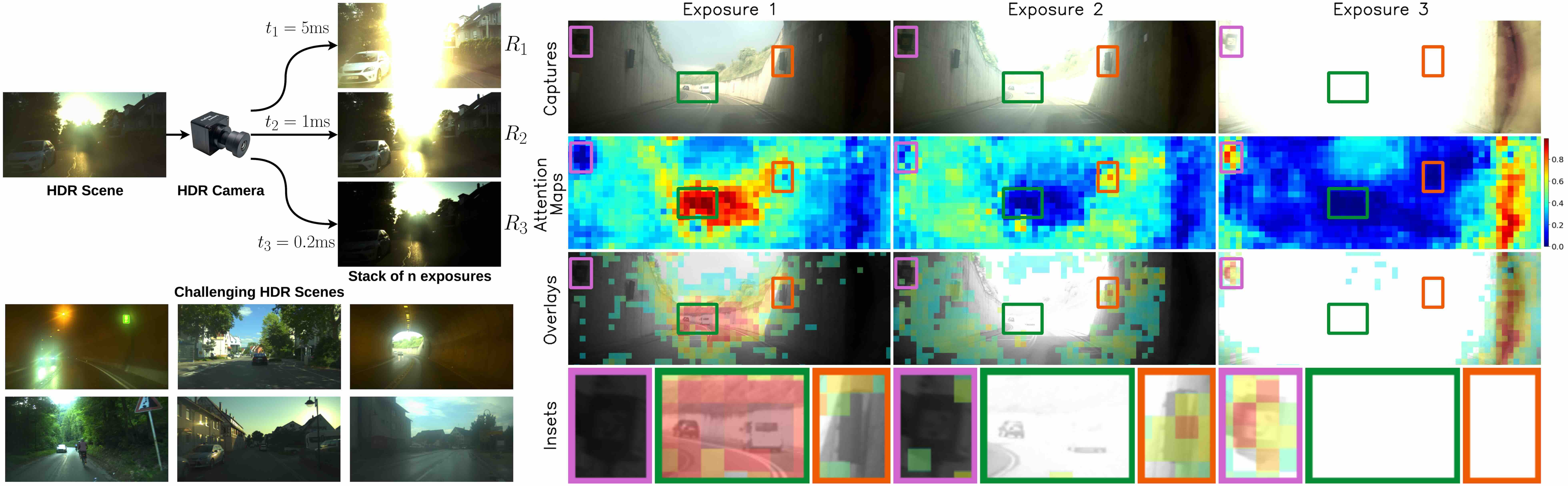
Computer vision in unconstrained outdoor scenarios must tackle challenging high dynamic range (HDR) scenes and rapidly changing illumination conditions. Existing methods address this problem with multi-capture HDR sensors and a hardware image signal processor (ISP) that produces a single fused image as input to a downstream neural network. The output of the HDR sensor is a set of low dynamic range (LDR) exposures, and the fusion in the ISP is performed in image space and typically optimized for human perception on a display. Preferring tonemapped content with smooth transition regions over detail (and noise) in the resulting image, this image fusion does typically not preserve all information from the LDR exposures that may be essential for downstream computer vision tasks. In this work, we depart from conventional HDR image fusion and propose a learned task-driven fusion in the feature domain. Instead of using a single companded image, we introduce a novel local cross-attention fusion mechanism that exploits semantic features from all exposures - learned in an end-to-end fashion with supervision from downstream detection losses. The proposed method outperforms all tested conventional HDR exposure fusion and auto-exposure methods in challenging automotive HDR scenarios.
Neural Exposure Fusion for High-Dynamic Range Object Detection
Emmanuel Onzon, Maximilian Bömer, Fahim Mannan, Felix Heide
CVPR 2024


Conventional HDR exposure fusion is done in image space, before object detection. We propose an alternative approach to HDR object detection, where multi-exposure captures are not merged on the sensor but fused in the feature domain. The proposed pipeline reasons on features from separate exposures, fusing them via an attention module. and relies on an attention module. This module, along with all the other pipeline components, is trained end-to-end driven by the detection loss.
Side-by-side comparison of conventional HDR Fusion in image space (top) and proposed Local Cross Attention Fusion in feature space (bottom) for challenging HDR scenes. Our neural fusion module recovers features from separate exposure streams, where the image region is well exposed to make its decision. In contrast, the fused HDR image misses details and local contrast resulting in false negatives and false positives. Final detections are overlaid over all Cross-Attention exposures.
Qualitative results of our proposed neural HDR object detection pipeline, that jointly learns exposure control, image processing, feature extraction, fusion and detection. Final detections are overlaid on the three processed exposure captures.
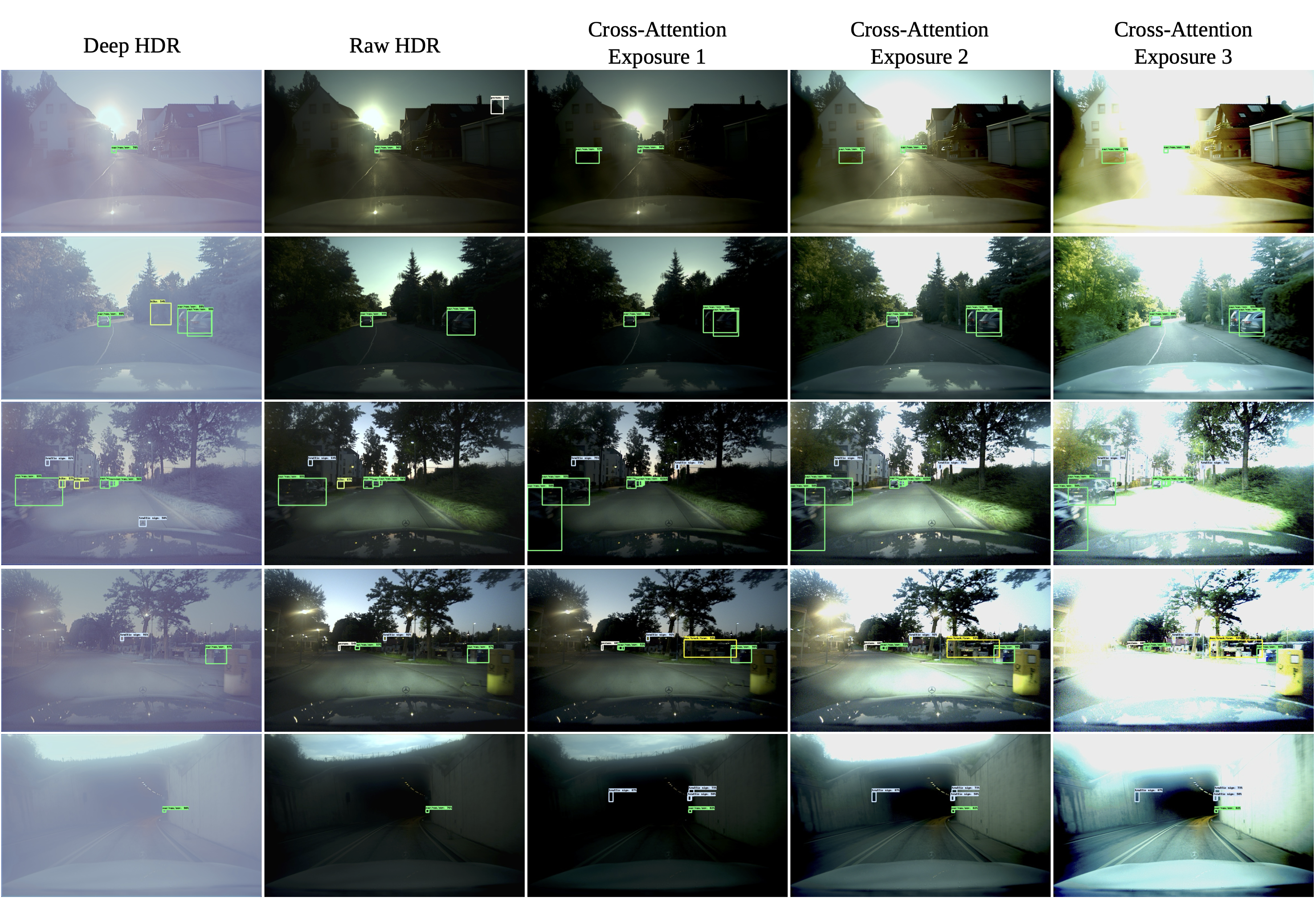
Qualitative comparison of the proposed Local Cross-Attention Fusion with the baseline methods Raw HDR and Deep HDR on challenging scenes. Final detections in the last three columns are overlaid over all Cross-Attention exposures.
Related Publications
[1] Emmanuel Onzon, Fahim Mannan and Felix Heide. Neural Auto Exposure for High-Dynamic Range Object Detection. The IEEE International Conference on Computer Vision (CVPR), 2021.
[2] Ali Mosleh, Avinash Sharma, Emmanuel Onzon, Fahim Mannan, Nicolas Robidoux and Felix Heide. Hardware-in-the-loop End-to-end Optimization of Camera Image Processing Pipelines. The IEEE International Conference on Computer Vision (CVPR), 2020.
[3] Nicolas Robidoux, Luis Eduardo García Capel, Dong-eun Seo, Avinash Sharma, Federico Ariza and Felix Heide. End-to-end High Dynamic Range Camera Pipeline Optimization. The IEEE International Conference on Computer Vision (CVPR), 2021.