ZeroScatter: Domain Transfer for Long Distance Imaging and Vision through Scattering Media
- Zheng Shi
- Ethan Tseng
- Mario Bijelic
-
Werner Ritter
- Felix Heide
CVPR 2021

Scattering media stemming from snow, rain, or fog significantly reduce the perceptible quality of RGB captures and impact downstream computer vision tasks such as object detection. Our proposed ZeroScatter reliably removes these scattering effects for unseen automotive scenes.
Adverse weather conditions, including snow, rain, and fog, pose a major challenge for both human and computer vision. Handling these environmental conditions is essential for safe decision making, especially in autonomous vehicles, robotics, and drones. Most of today's supervised imaging and vision approaches, however, rely on training data collected in the real world that is biased towards good weather conditions, with dense fog, snow, and heavy rain as outliers in these datasets. Without training data, let alone paired data, existing autonomous vehicles often limit themselves to good conditions and stop when dense fog or snow is detected. In this work, we tackle the lack of supervised training data by combining synthetic and indirect supervision. We present ZeroScatter, a domain transfer method for converting RGB-only captures taken in adverse weather into clear daytime scenes. ZeroScatter exploits model-based, temporal, multi-view, multi-modal, and adversarial cues in a joint fashion, allowing us to train on unpaired, biased data. We assess the proposed method on in-the-wild captures, and the proposed method outperforms existing monocular descattering approaches by 2.8 dB PSNR on controlled fog chamber measurements.
ZeroScatter: Domain Transfer for Long Distance Imaging and Vision through Scattering Media
Zheng Shi*, Ethan Tseng*, Mario Bijelic*, Werner Ritter, Felix Heide
(* indicates equal contribution)
CVPR 2021
Video Summary
We train our network using a novel combination of training cues that promote high-contrast, scatter-free, jitter-free results on unseen real-world scenes. We employ model-based supervision using cycle training which is facilitated by a robust adverse weather model, multi-modal supervision in the form of gated images for training on real heavy weather scenes, and consistency supervision in the form of temporal and stereo losses.
Selected Results
Controlled Experimental Evaluation.
Qualitative performance comparison on controlled fog chamber measurements. Our proposed approach significantly reduces scattering media present in the scene and most closely resembles the daytime stylized target image.
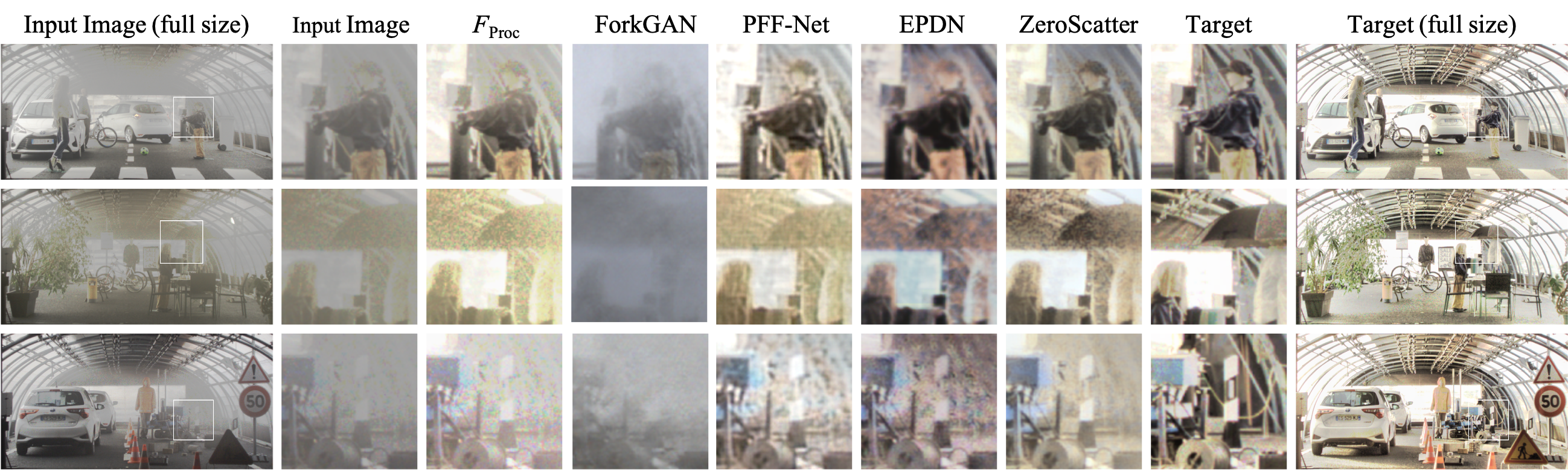
Real-world Experimental Evaluation.
Real-world data qualitative comparisons. Our proposed approach significantly reduces scattering present in the scene and reveals object in long distance, such as the house and trees in the top two examples above. Compared to EPDN and PFF-Net, ZeroScatter is able to produce images with better contrast and less noise. ZeroScatter is able to remove snowflakes in the 3rd and 4th examples and sensor noise in the 5th and 6th examples.
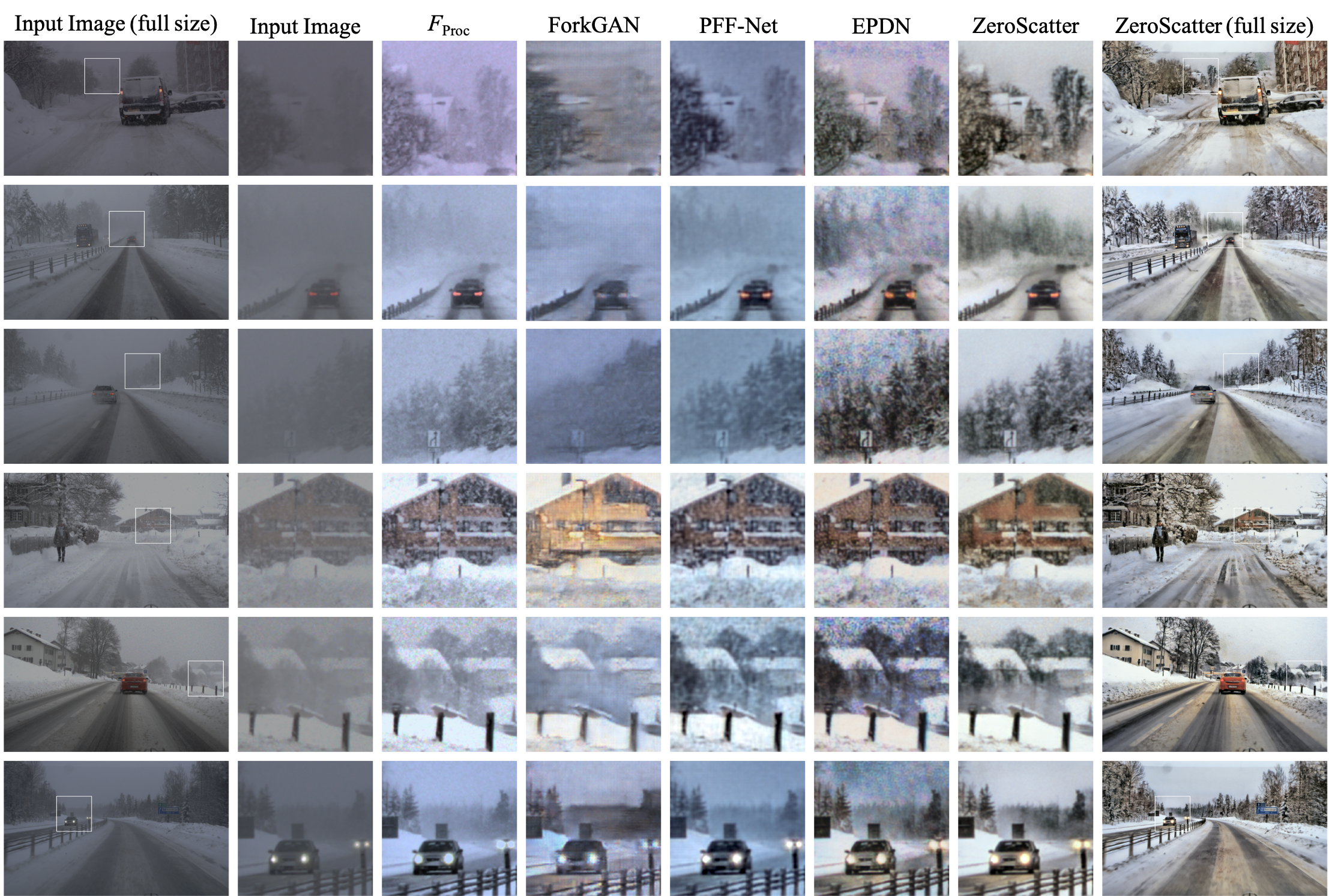
De-scattering for Object Detection.
Object detection qualitative comparisons. Each row shows the detector’s performance on each method’s output. White boxes are the ground truth labels; red boxes and the number in the corner are detection box output and the corresponding confidence respectively. Compared to the baseline methods, ZeroScatter allows the detector to detect more objects at far distances, such as in the first three examples, and with higher confidence, such as in the first and fourth examples.
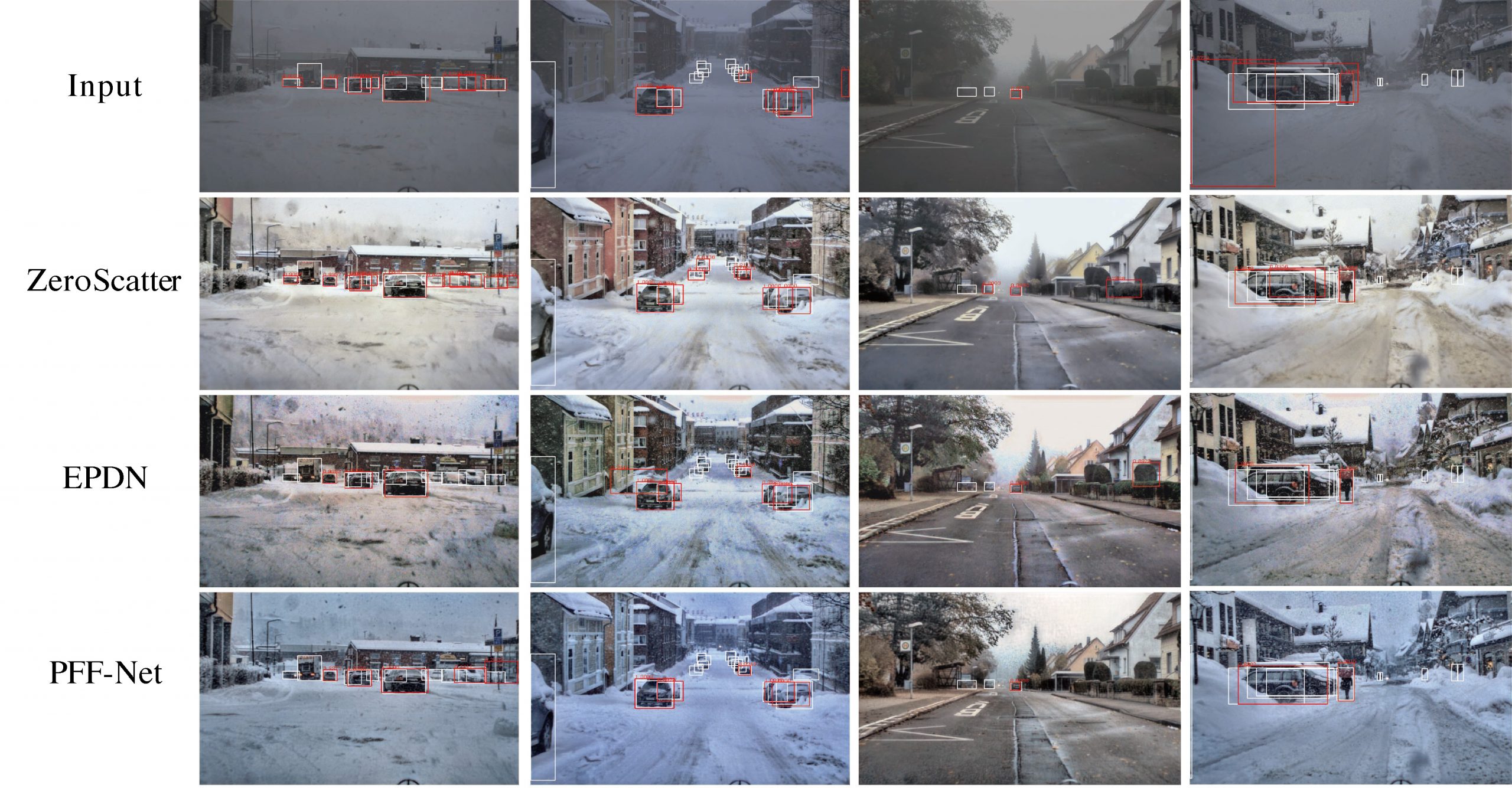