Spiking Transformers for Event-based Single Object Tracking
-
Jiqing Zhang
- Bo Dong
-
Haiwei Zhang
-
Jianchuan Ding
- Felix Heide
-
Baocai Yin
- Xin Yang
CVPR 2022
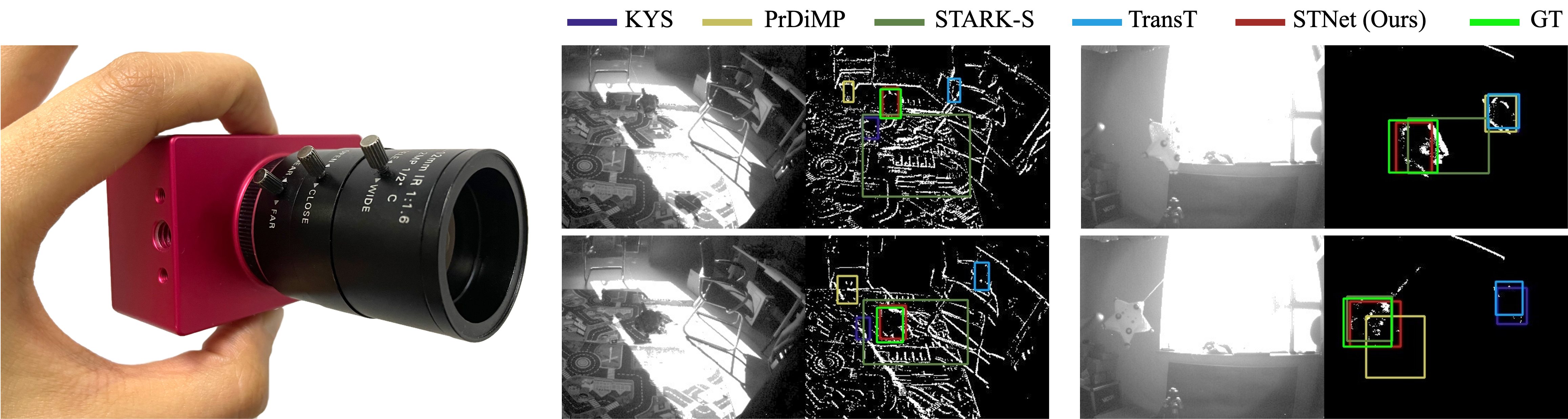
Intensity Frame Event Frame Intensity Frame Event Frame
Event-based Camera Severe Camera Motion High Dynamic Range
We introduce a spiking transformer network for single object tracking from event frames, STNet, that is capable of fusing temporal and spatial cues encoded in the events. STNet outperforms existing tracking methods on the FE240hz dataset. We compare here against the tracking methods KYS, PrDiMP, STARK-S, and TransT. STNet offers the best performance even in challenging HDR scenes and under severe camera motion.
Event-based cameras bring a unique capability to tracking, being able to record intensity changes with high temporal resolution and high dynamic range. These imagers capture such events asynchronously which implicitly encode rich temporal and spatial information. However, effectively extracting this information from events remains an open challenge. In this work, we propose a spiking transformer network, STNet, for single object tracking. STNet dynamically extracts and fuses information in both the temporal and spatial domains. In particular, the proposed architecture features a transformer module to provide global spatial information and a spiking neural network (SNN) module for extracting temporal cues. The spiking threshold of the SNN module is dynamically adjusted based on the statistical cues of the spatial features, which we find essential in providing robust SNN features. We fuse both feature branches dynamically with a novel cross-domain attention fusion algorithm. Extensive experiments on three event-based datasets, FE240hz, EED, and VisEvent validate that the proposed STNet outperforms existing state-of-the-art methods in both tracking accuracy and speed by a significant margin.
Paper
Jiqing Zhang, Bo Dong, Haiwei Zhang, Jianchuan Ding, Felix Heide, Baocai Yin, and Xin Yang
Spiking Transformers for Event-based Single Object Tracking
CVPR 2022
Spiking Transformer Network (STNet)
Exploiting events captured by an event-based camera for object tracking requires tackling the following two challenges: 1) The event domain provides rich temporal information but in an asynchronous manner. Existing deep-learning-based approaches convert these asynchronous events to conventional intensity images for extracting spatial features. However, during the process, the original temporal information is partially lost. 2) Even if temporal information can be extracted from the events, dynamically fusing temporal and spatial cues remains challenging. In this work, we propose a spiking transformer network (STNet) for single object tracking in the event domain, consisting of two key components: SNNformer feature extractor (SFE) and temporal-spatial feature fusion (TSFF), see paper.
Qualitative Experiments on FE240hz
We qualitatively demonstrate the benefits of the proposed STNet when compared to SOTA trackers, including KYS, PrDiMP, STARK-S, and TransT, under four different degraded conditions: scenes with a) other objects similar to the object being tracked (SOSOBT); b) severe camera motion; c) strobe light, and d) high dynamic range.
Quantitative Experiments on FE240hz
We report the tracking performance of the top five methods on FE240hz in the following degraded conditions: (1) scenes with objects similar to the object being tracked; (2) severe camera motion; (3) scenes illuminated with a strobe light (i.e., periodically turned on/off lights), and (4) high dynamic range (HDR) scenes.
Here, tracking performance is quantitatively measured by the following two metrics: success rate and precision rate. The success rate focuses on the frame where the overlap between ground truth and predicted bounding box is larger than a threshold; the precision rate counts the frame on which the center distance between ground truth and predicted bounding box is within a given threshold. We use the area under the curve as the representative success rate (RSR). The representative precision rate (RPR) is defined as a PR score associated with a 20-pixel threshold. As shown in (a), our approach fares best in all degraded conditions. In (b), we report the RPR and RSR with respect to the tracking speed of the top five methods on the FE240hz dataset.