Learned Hardware-in-the-loop Phase Retrieval for Holographic Near-Eye Displays
- Praneeth Chakravarthula
- Ethan Tseng
-
Tarun Srivastava
- Henry Fuchs
- Felix Heide
SIGGRAPH Asia 2020
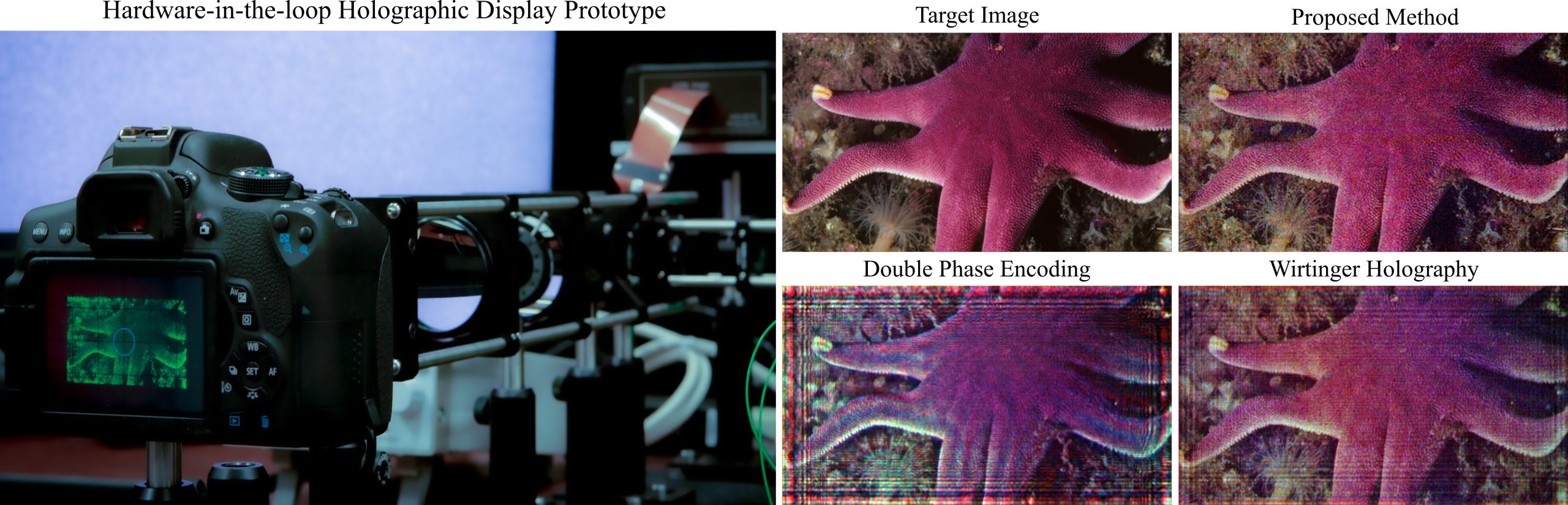
Holographic displays often show poor image quality due to severe real world deviations in the light propagation compared to the simulated “ideal” light propagation model. We built a holographic display-camera setup (left) to generate data that is used to train a neural network for approximating the unknown light propagation in a real display and the resulting aberrations. We then use this trained network to compute phase holograms that compensate for real world aberrations in a hardware-in-the-loop fashion. This allows us to supervise the display states with unknown light propagation just by observing image captures of the display prototype (left). Compared to holographic images captured using state-of-the-art methods, Double Phase Encoding [Maimone et al. 2017] (bottom center) and Wirtinger Holography [Chakravarthula et al. 2019] (bottom right), the proposed method (top right) produces images on real hardware that are aberration-free and close to the target image (top center).
Holography is arguably the most promising technology to provide wide field-of-view compact eyeglasses-style near-eye displays for augmented and virtual reality. However, the image quality of existing holographic displays is far from that of current generation conventional displays, effectively making today's holographic display systems impractical. This gap stems predominantly from the severe deviations in the idealized approximations of the "unknown" light transport model in a real holographic display, used for computing holograms.
In this work, we depart from such approximate "ideal" coherent light transport models for computing holograms. Instead, we learn the deviations of the real display from the ideal light transport, from the images measured using a display-camera hardware system. After this unknown light propagation is learned, we use it to compensate for severe aberrations in real holographic imagery. The proposed hardware-in-the-loop approach is robust to spatial, temporal and hardware deviations, and improves the image quality of existing methods qualitatively and quantitatively in SNR and perceptual quality. We validate our approach on a holographic display prototype and show that the method can fully compensate unknown aberrations and erroneous and non-linear SLM phase delays, without explicitly modeling them. As a result, the proposed method significantly outperforms existing state-of-the-art methods in simulation and experimentation -- just by observing captured holographic images.
Paper
Praneeth Chakravarthula, Ethan Tseng, Tarun Srivastava, Henry Fuchs, Felix Heide
Learned Hardware-in-the-loop Phase Retrieval for Holographic Near-Eye Displays
SIGGRAPH Asia 2020
Video Summary
Selected Results
The exact coherent light transport is unknown in real holographic displays. Holograms are typically computed assuming an ideal light transport and this causes severe aberrations in holographic images. Computing holograms in a display-camera hardware-in-the-loop fashion can mitigate aberrations but is not practical for (near-eye) display applications.
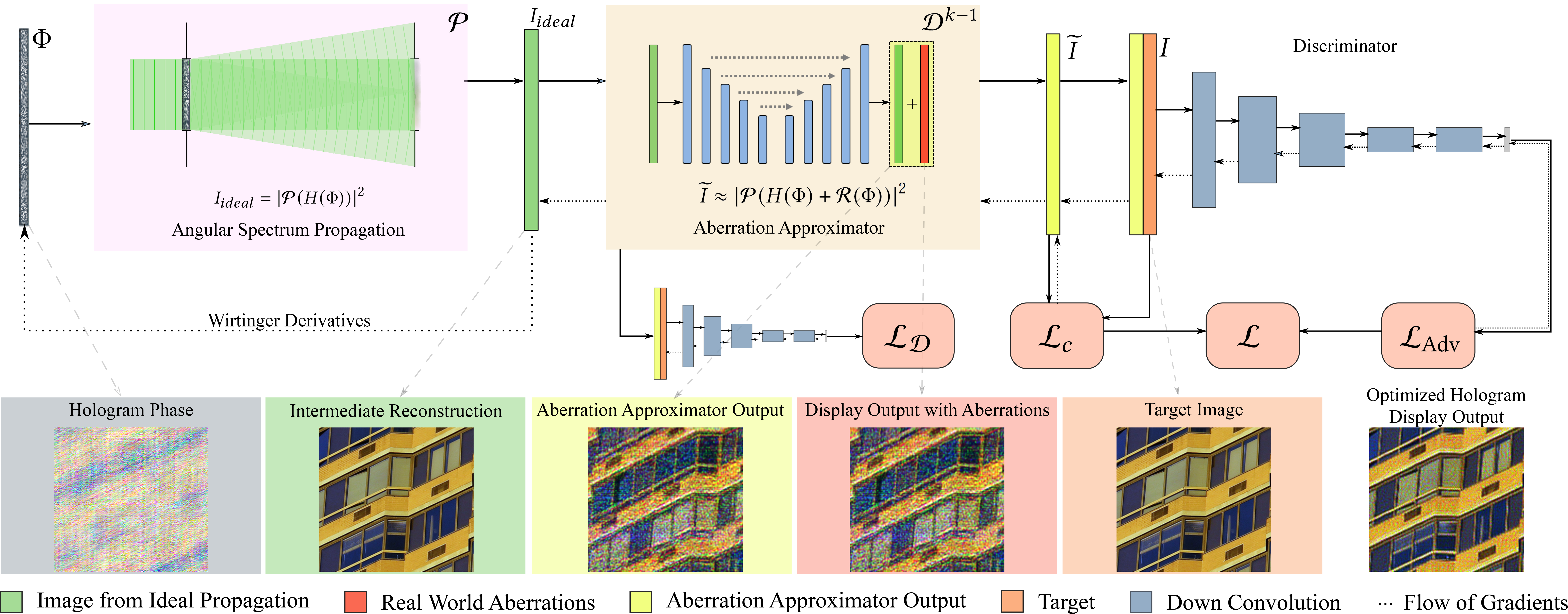
Aberration Approximator
We learn the unknown light transport by training a neural network on hardware-in-the-loop holographic image captures. We then use this trained network as a substitute for real holographic display and compensate for severe aberrations. Our method neither requires explicit modeling of the source of errors nor an online camera for hologram optimization.
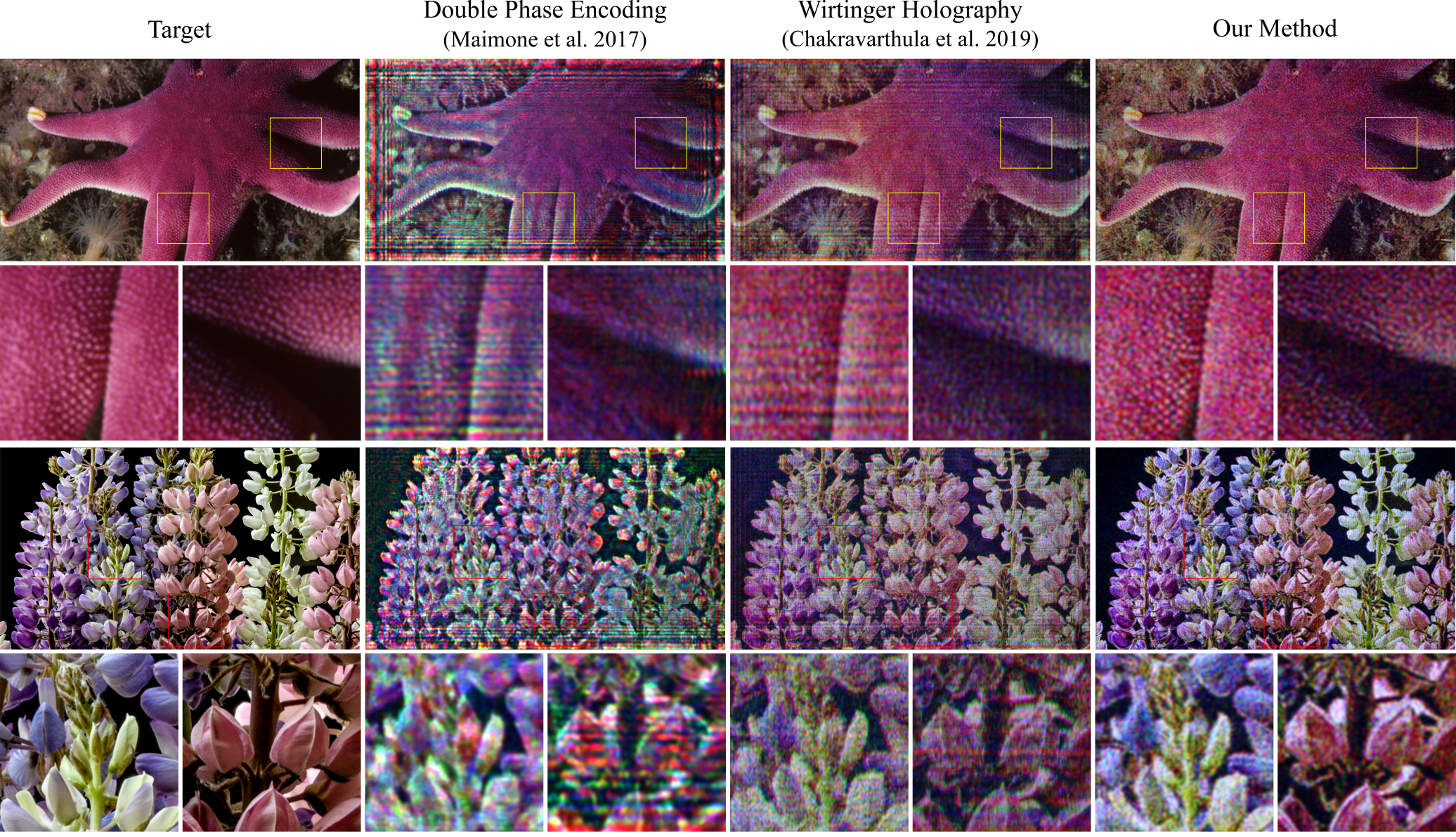
High-Quality Holograms
Holograms computed using our "learned hardware-in-the-loop phase retrieval" eliminates severe aberrations and show an improved quality, enhanced resolution and remarkable contrast, with black regions truly being black as shown below. We achieve all of this in a holographic display, perhaps for the first time to the best of our knowledge.
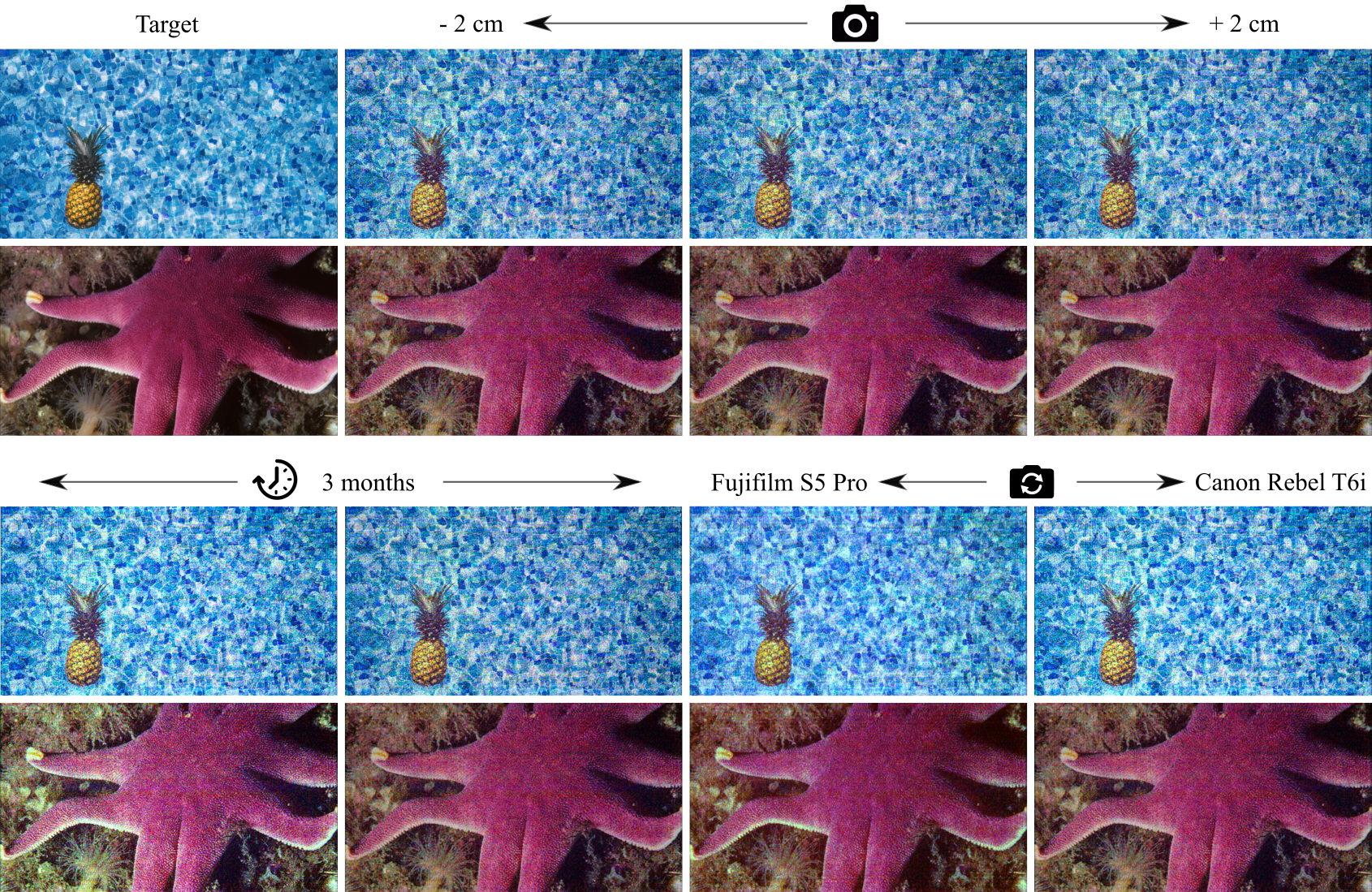
Robustness to deviations
Our method is robust to spatial, temporal and hardware deviations. Here, we show captured images from holograms computed using our method for different conditions. The images captured over a period of 3 months, during which the hardware was disassembled, moved to a different place and reassembled, due to the COVID-19 pandemic, shows the promise of our method to generalize per device type of future display eyeglasses. The disparity in the brightness and color is due to the differences in the laser power and we note that these are not fundamental to the proposed method.
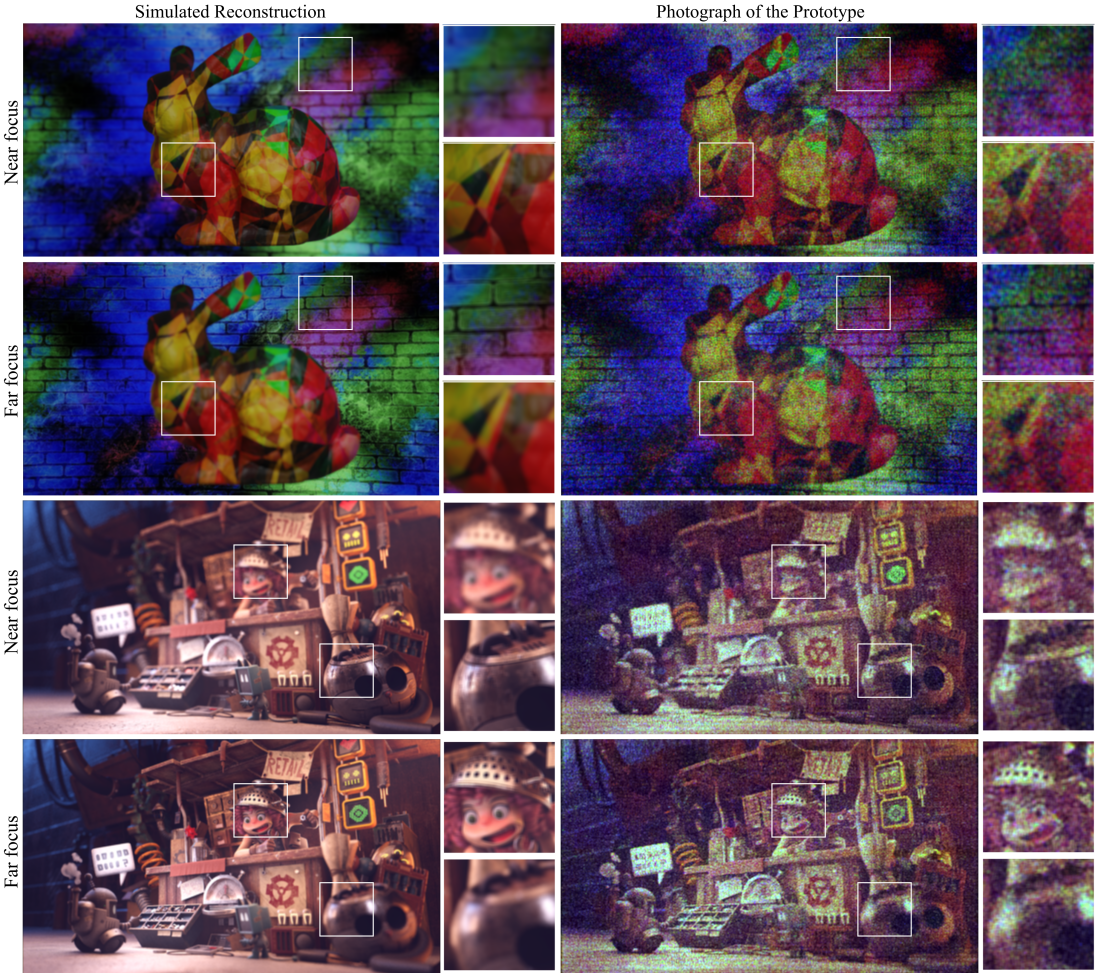
3D Holograms
Our method can also be extended to 3D holograms.