Glass Segmentation using Intensity and Spectral Polarization Cues
- Haiyang Mei
- Bo Dong
-
Wen Dong
-
-
Jiaxi Yang
- Seung-Hwan Baek
- Felix Heide
- Pieter Peers
-
Xiaopeng Wei
- Xin Yang
CVPR 2022
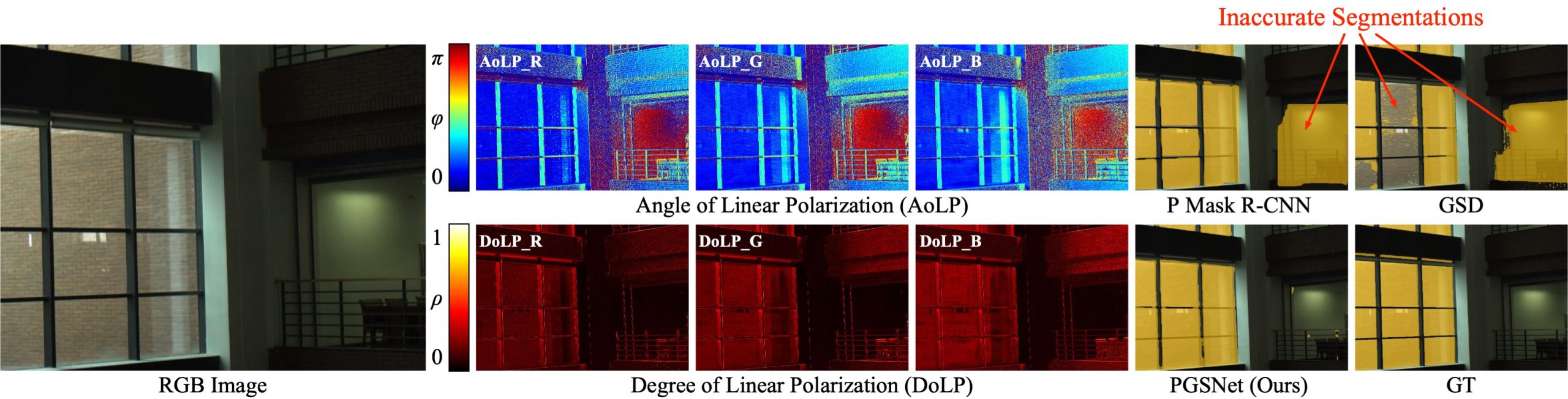
We introduce a glass segmentation network (PGSNet) that relies on both trichromatic (RGB) intensities and trichromatic linear polarization cues captured in a single photograph without making any assumption on the polarization state of the illumination. We show here glass segmentations obtained with the RGB-only method GSD and monochromatic polarization method P Mask R-CNN compared to the proposed PGSNet. Without spectral polarization cues, existing methods often fail to cleanly separate the non-glass regions with a similar appearance.
Transparent and semi-transparent materials pose significant challenges for existing scene understanding and segmentation algorithms due to their lack of RGB texture which impedes the extraction of meaningful features. In this work, we exploit that the light-matter interactions on glass materials provide unique intensity-polarization cues for each observed wavelength of light. We introduce a glass segmentation network that leverages both trichromatic (RGB) intensities as well as trichromatic linear polarization cues from a single photograph captured without making any assumption on the polarization state of the illumination. Our network dynamically fuses and weights both the trichromatic color and polarization cues with a global-guidance and multi-scale self-attention module. In addition, we exploit global cross-domain contextual information to achieve robust segmentation. We train and extensively validate our segmentation method on a new large-scale RGB-Polarization dataset (RGBP-Glass), and demonstrate that our method outperforms state-of-the-art segmentation approaches by a significant margin.
Paper
Haiyang Mei, Bo Dong, Wen Dong, Jiaxi Yang, Seung-Hwan Baek, Felix Heide, Pieter Peers, Xiaopeng Wei, and Xin Yang
Glass Segmentation using Intensity and Spectral Polarization Cues
CVPR 2022
Qualitative comparison to existing glass segmentation methods
1. Bathroom scene (1st example): reflections on the glass surfaces share the texture of the wall. Nevertheless, our method is able to accurately segment the scene.
2. Glass in metal door-frame (2ed example): all methods except PGSNet and Trans2Seg confuse the metal surface for glass.
3. “Invisible” glass (3rd and 4th example): even though the surface is invisible in the RGB intensity image, we observe strong cues from both spectral polarization cues. Our method succeeds because of the proposed dynamic context-aware attention-based fusing strategy.
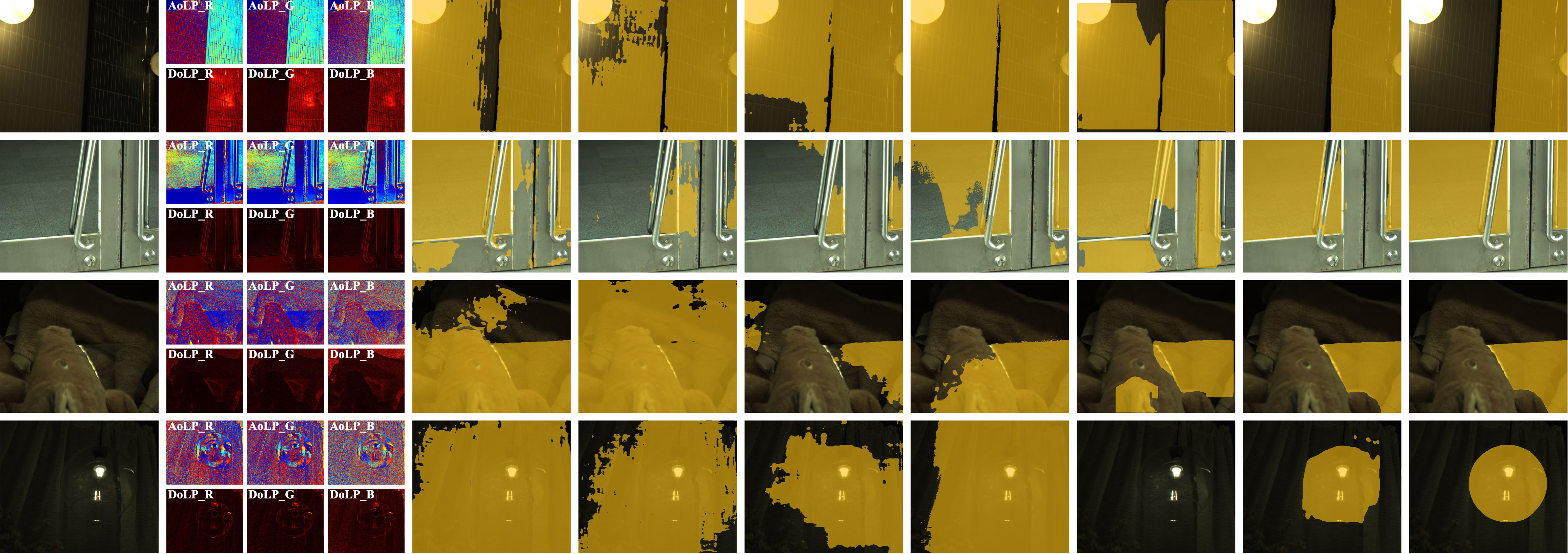
RGB Image Trichromatic Polarization Cues GDNet TransLab Trans2Seg GSD P Mask R-CNN PGNet (Ours) GT
Dynamic Scene Results
We provide additional qualitative results of PGSNet on dynamic scenes. Even though the proposed approach is not designed for dynamic scenes, the method is temporally stable. RGB AoLP/DoLP R AoLP/DoLP G AoLP/DoLP B Prediction
RGB-P Glass Segmentation Dataset
We collect a large-scale polarization glass segmentation dataset, which we dub RGBP-Glass, using a trichromatic polarizer-array camera (LUCID PHX050S) that records four different linear-polarization directions (0, 45, 90, and 135 degrees) for each color channel (i.e., R, G, and B). RGBP- Glass contains 4,511 RGB intensity and corresponding pixel-aligned trichromatic AoLP and DoLP images with manually annotated pixel-level accurate reference glass masks and associated bounding boxes. Each image in RGBP-Glass contains at least one in-the-wild glass object. To ensure diversity of scenes, we capture the dataset from different locations, view angles, lighting conditions, types of glass, and shapes of glass.
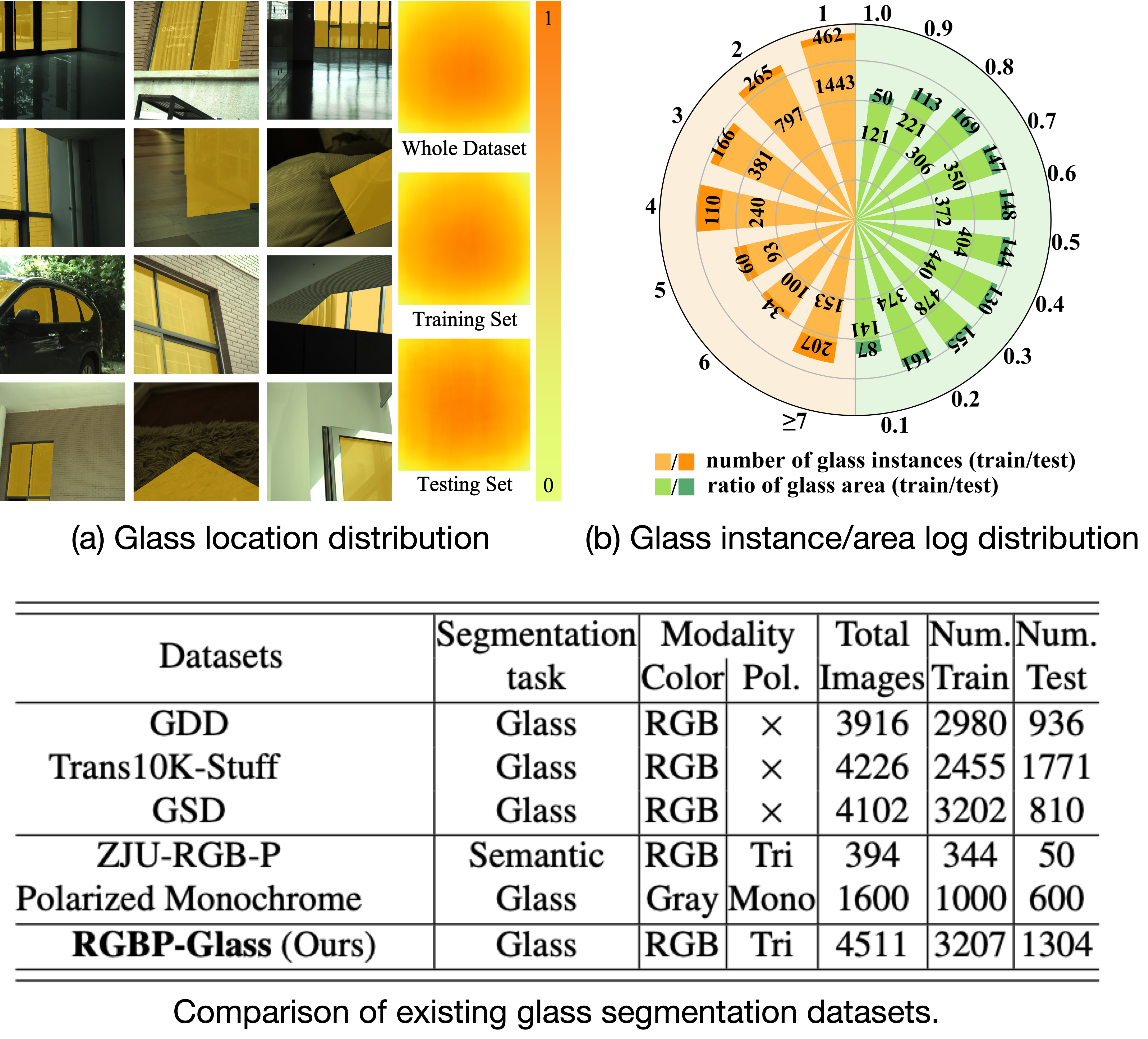
Polarization Glass Segmentation Network (PGSNet)
The proposed Polarization Glass Segmentation Network (PGSNet) fuses multimodal intensity and polarization measurements by leveraging both local and global contextual information. PGSNet follows the encoder-decoder architecture illustrated below. During encoding, an early dynamic attention module (EDA) estimates global scaling weights for balancing the different color channels within each of the trichromatic angle of linear polarization (AoLP) and degree of linear polarization (DoLP). Next, the weighted trichromatic AoLP and DoLP along with the RGB intensity image are passed into three separate Conformer branches for feature extraction. The goal of the Conformer stage is to balance differences between glass and non-glass objects within each of the different sources. In the final encoding step, we employ a novel Dynamic Multimodal Feature Integration (DMFI) module to dynamically fuse together the extracted local features from the three input sources (i.e., RGB, AoLP, and DoLP) guided by the global features. On the decoder side, we introduce a novel Global Context Guided Decoder (GCGD) that employs an Attention Enhancement (AE) module to dynamically provide global guidance using multimodal global features from the three Conformer branches.
Related Publications
[1] Seung-Hwan Baek and Felix Heide Polarimetric Spatio-Temporal Light Transport Probing. ACM SIGGRAPH Asia, 2021