Seeing Around Street Corners: Non-Line-of-Sight Detection and Tracking In-the-Wild Using Doppler Radar
-
Nicolas Scheiner
-
Florian Kraus
- Fangyin Wei
-
Buu Phan
-
Fahim Mannan
-
Nils Appenrodt
-
Werner Ritter
-
Jürgen Dickmann
-
Klaus Dietmayer
-
Bernhard Sick
- Felix Heide
CVPR 2020
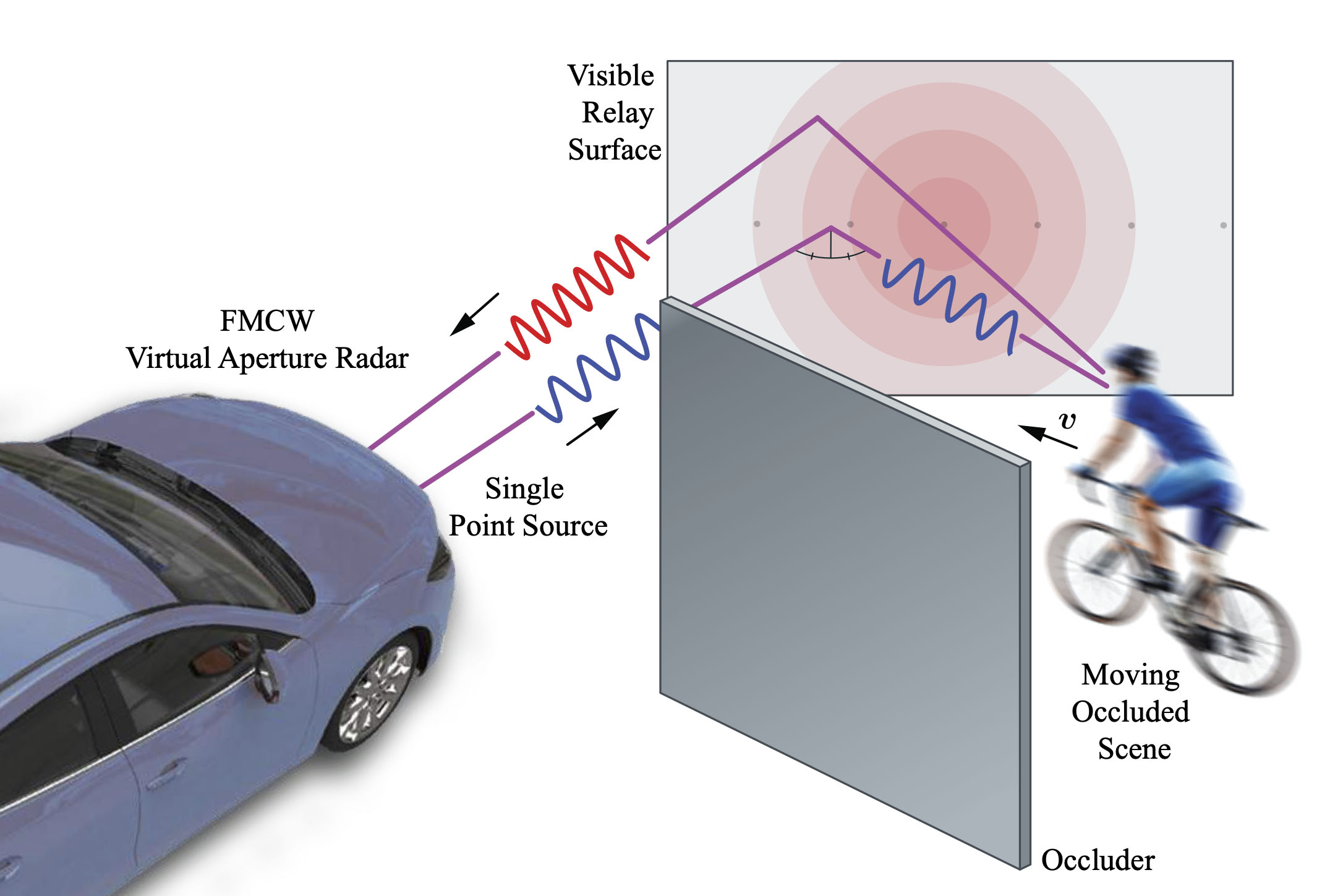
We demonstrate that it is possible to recover moving objects outside the direct line of sight in large automotive environments from Doppler radar measurements. Using static building facades or parked vehicles as relay walls, we jointly classify, reconstruct, and track occluded objects. Left: Illustration of the non-line-of-sight doppler image formation. Right: Joint detection and tracking results of the proposed model.
Paper
Nicolas Scheiner, Florian Kraus, Fangyin Wei, Buu Phan, Fahim Mannan, Nils Appenrodt,
Werner Ritter, Jürgen Dickmann, Klaus Dietmayer, Bernhard Sick, Felix Heide
Seeing Around Street Corners: Non-Line-of-Sight Detection and Tracking In-the-Wild Using Doppler Radar
CVPR 2020
Dataset
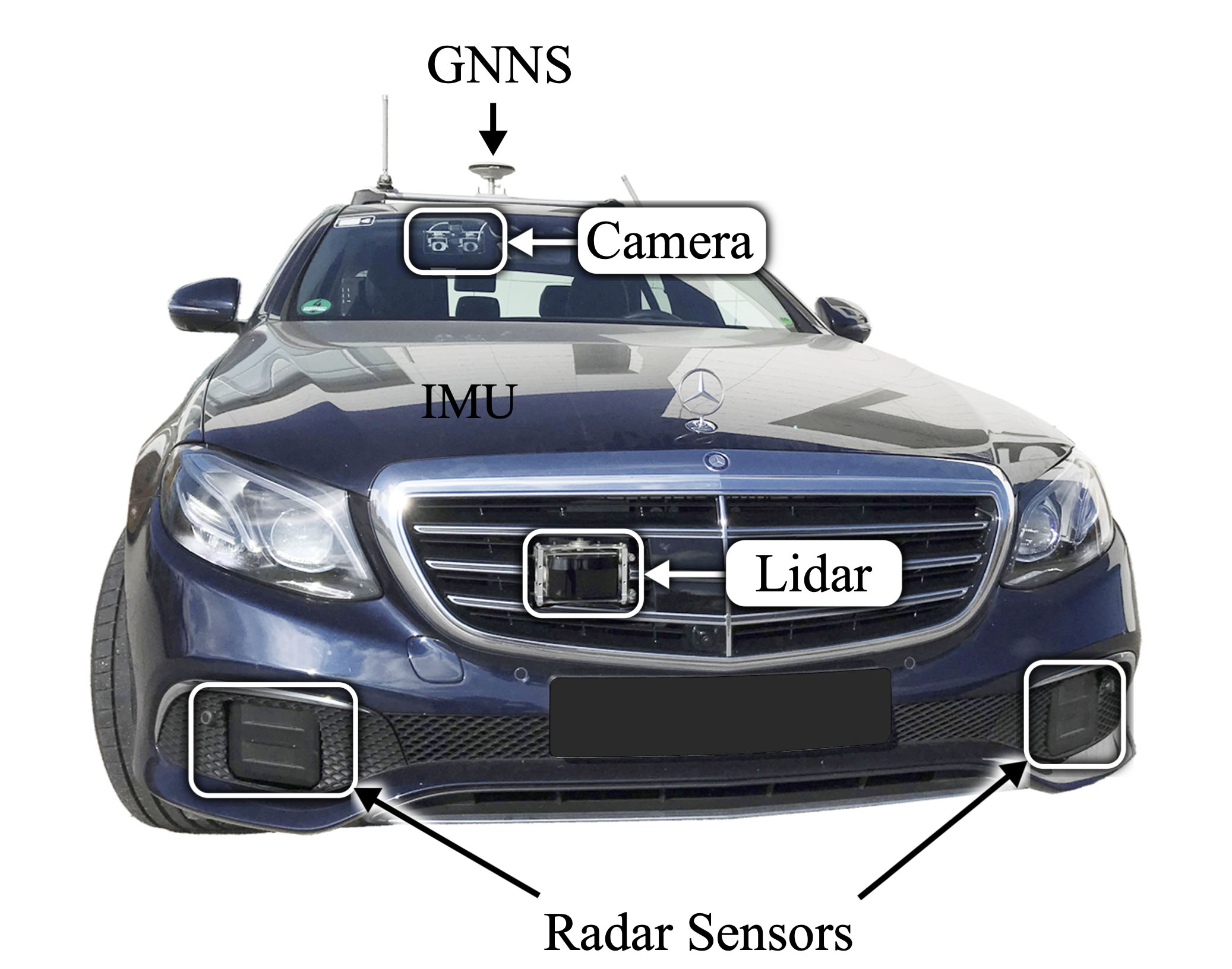
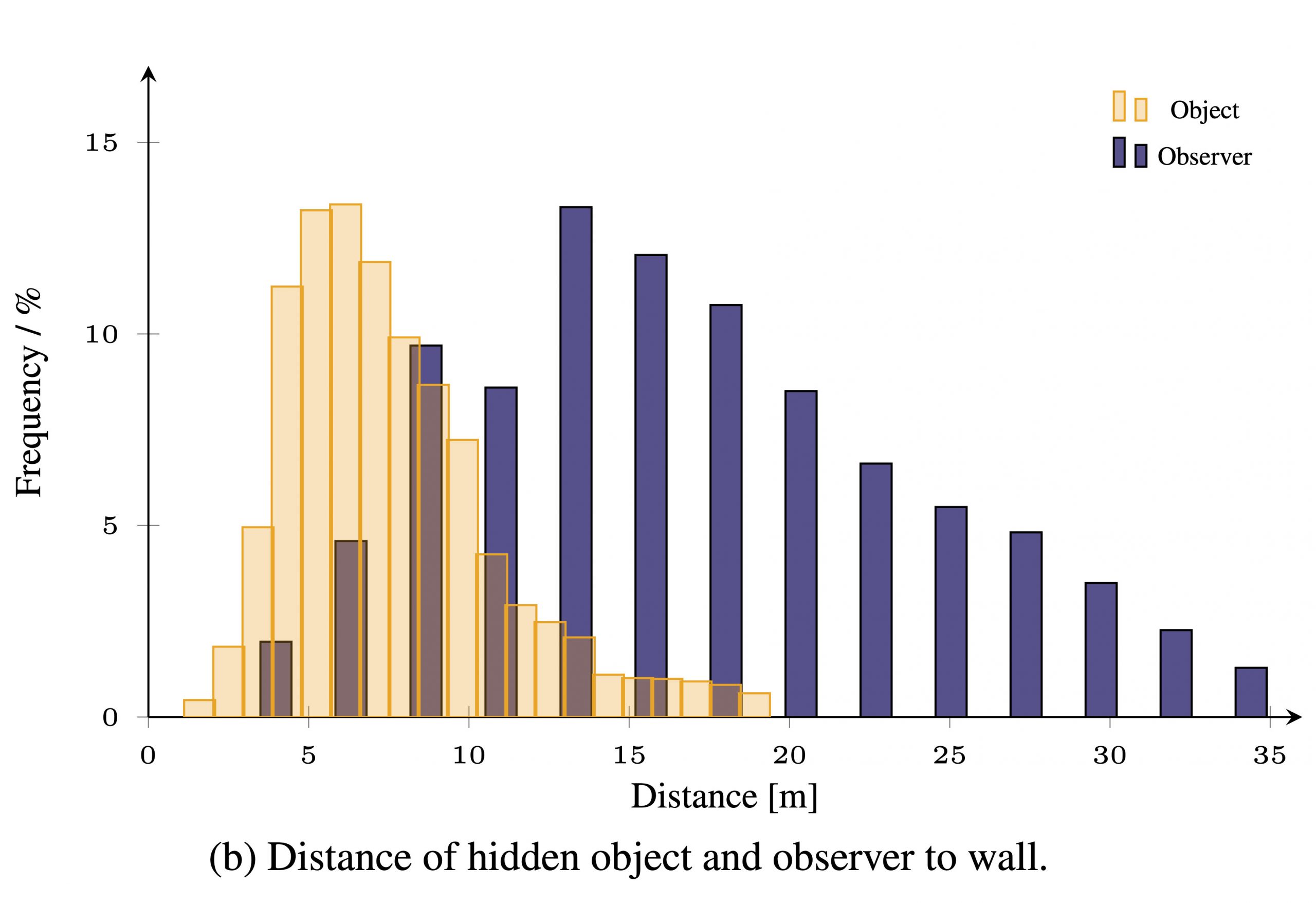
Validation and Training Dataset Acquisition and Statistics.
(a) Prototype vehicle with measurement setup. To acquire training data in an automated fashion we use GNSS and IMU for a full pose estimation of egovehicle and the hidden vulnerable road users.
(b) Hidden object and observer distances to the relay wall are in a wide range.
The following sample camera images including the (later on) hidden object. We show wide range of different types of relay walls appearing in this dataset.
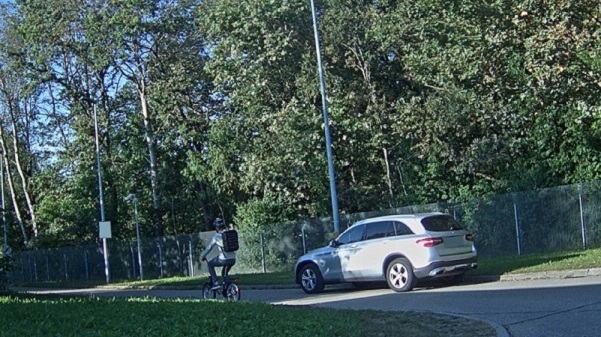
Single Car
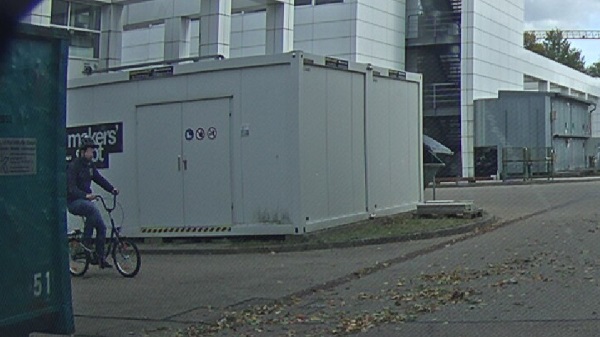
Mobile Office
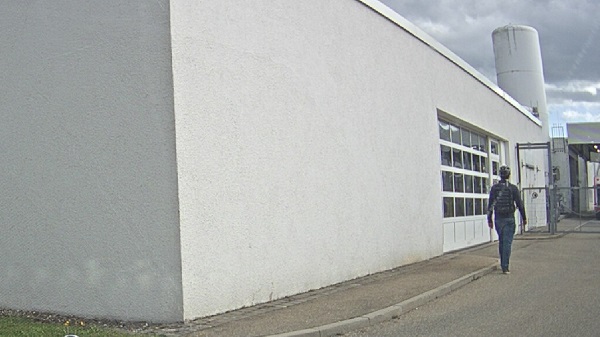
House Corner
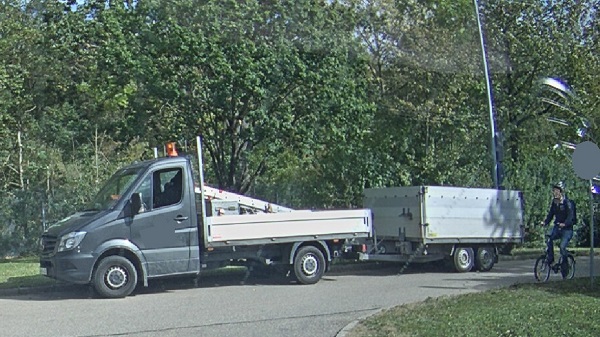
Van
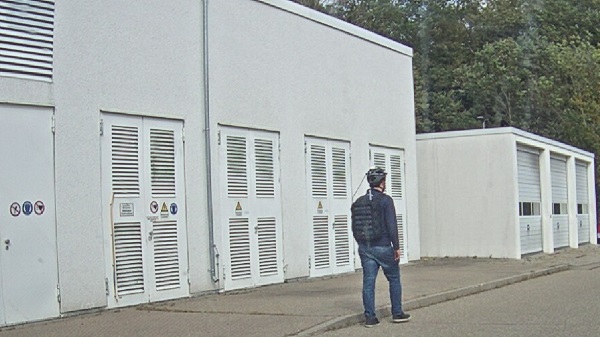
Utility Access
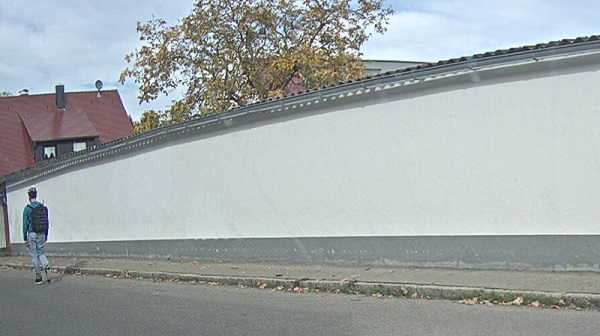
Garden Wall
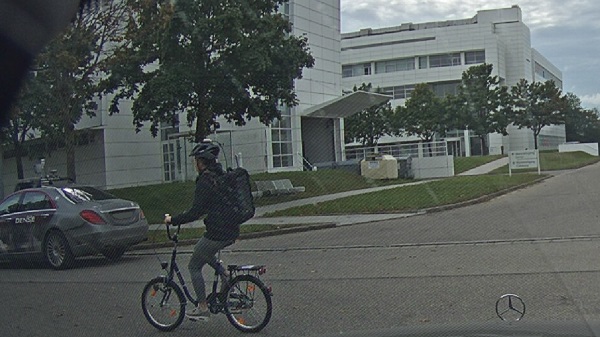
Three Cars
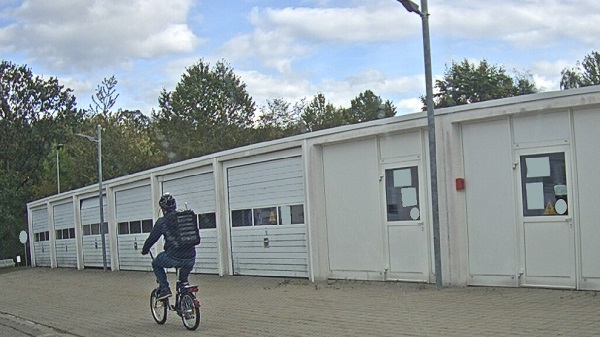
Garage Doors
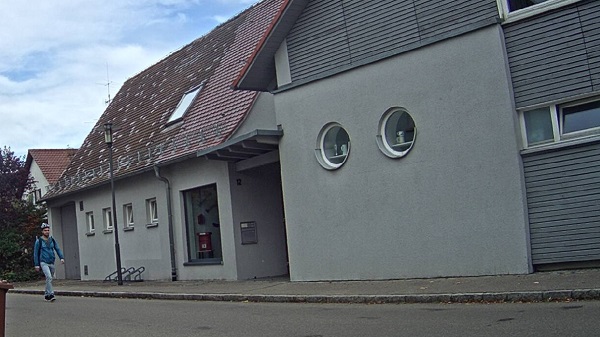
House Facade
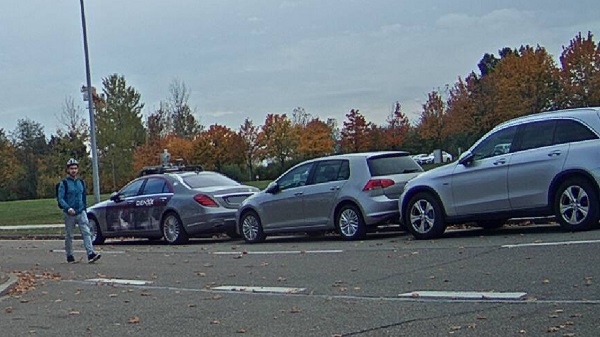
Three Cars
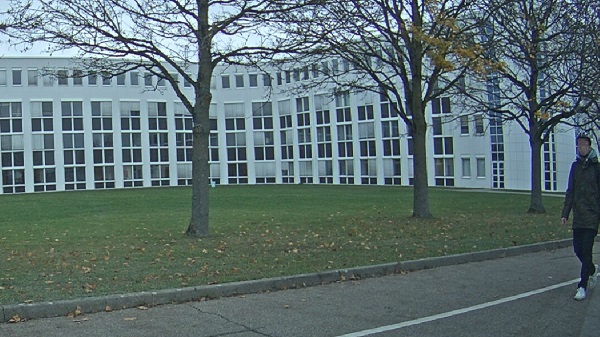
Curbstone
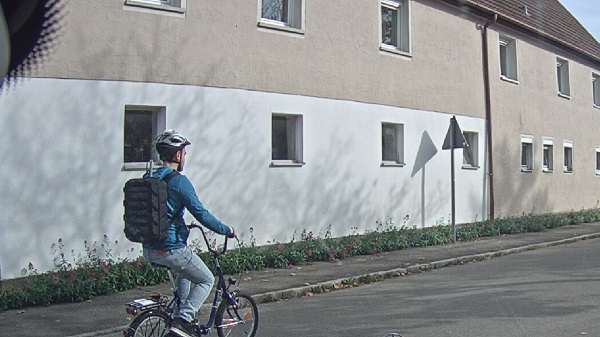
House Facade
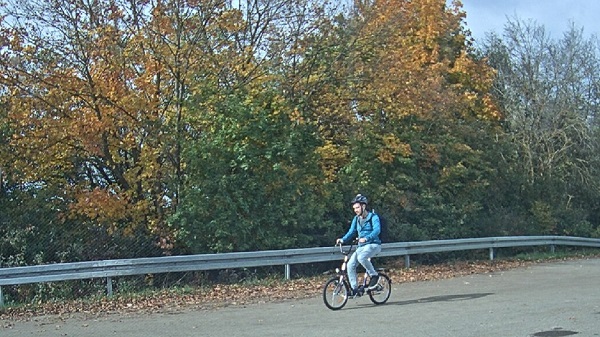
Guard Rail
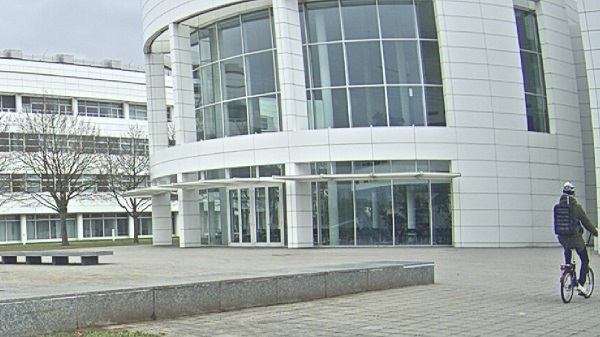
Marble Wall
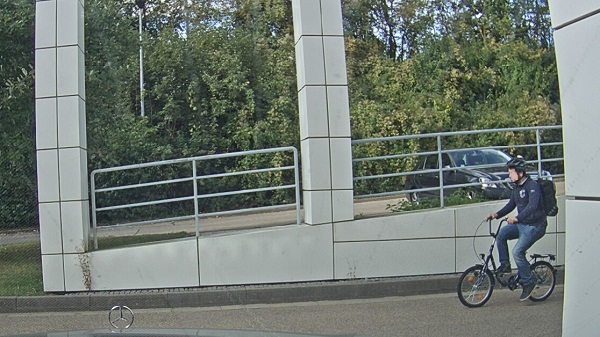
Building Exit
NLOS training and evaluation data set for large outdoor scenarios.
We capture a total of 100 sequences in-the-wild automotive scenes with 21 different scenarios. We split the dataset into non-overlapping training and validation sets, where the validation set consists of four scenes with 20 sequences and 3063 frames.
Selected Results
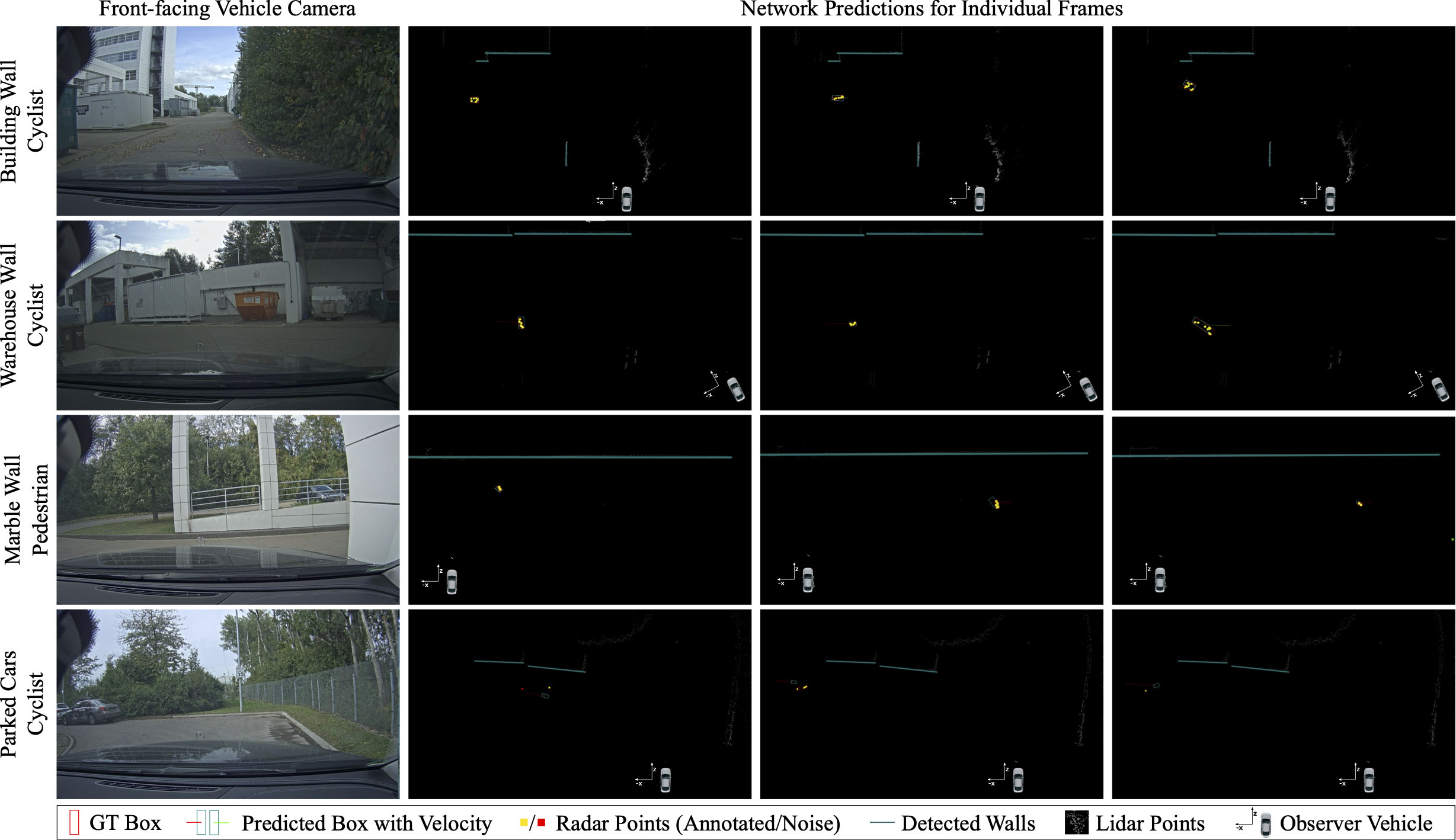
Joint detection and tracking results.
Joint detection and tracking results for automotive scenes with different relay wall type and object class in each row. The first column shows the observer vehicle front-facing camera view. The next three columns plot BEV radar and lidar point clouds together with bounding box ground truth and predictions. NLOS velocity is plotted as line segment from the predicted box center: red and green corresponds to moving towards and away from the vehicle.
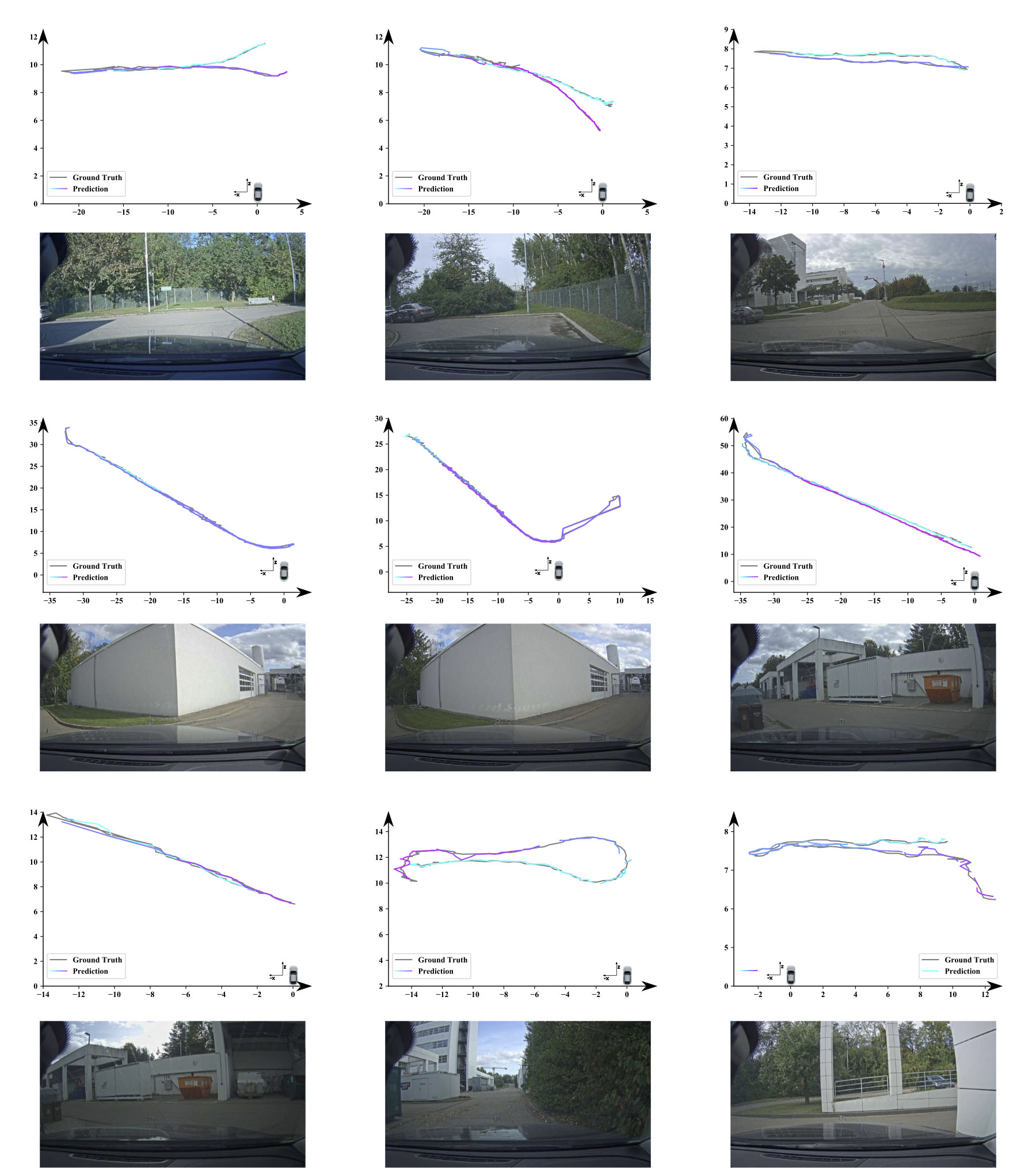
Tracking trajectories for both training and testing data sets.
Here we show nine scenes in total. The top-middle scene and the last three scenes are from the testing data set. For each scene, the first row is the trajectory and the second row is the front-facing vehicle camera. We can see a variety of wall types, trajectories and viewpoints of the observing vehicles. The predictions consist of segments, with each corresponding to a different tracking ID visualized in different colors.