Cross-spectral Gated-RGB Stereo Depth Estimation
CVPR 2024 (Highlight)
We propose Gated RCCB Stereo, a novel stereo-depth estimation method that is capable of exploiting multi-modal multi-view depth cues from RCCB and gated stereo images. We combine complementary cross-spectral features within an iterative stereo matching network with our proposed cross-spectral matching layer, utilizing intermediate depth estimations for accurate feature-registration. The proposed method achieves accurate depth at long ranges, outperforming the next best existing method by 39% for ranges of 100 to 220m in MAE on accumulated LiDAR ground-truth.
Paper
Cross-Spectral Depth Estimation from RGB and NIR-Gated Stereo Images
Samuel Brucker, Stefanie Walz, Mario Bijelic, Felix Heide
CVPR 2024
Cross-Spectral Gated and RCCB Imaging
Below, example images of both image modalities (left) are shown next to the sensor setup used for collecting the data. RCCB cameras (left top row) capture 8 Mpix passive RGB images. Gated cameras (left bottom row) record Time-of-Flight data of a scene by combining active flash illumination and analog gated readout. Both sensors are complementary, with distinct strengths depending on the scenario. RCCB cameras excel in daylight (a) with high dynamic range, resolution and color. At night (b, c), gated images (gated slices here RGB-color coded by mapping each slice to one RGB color) provide strong depth cues and maintain consistent scene illumination through active illumination. This work integrates both modalities to estimate depth accurately in all ambient illumination conditions.
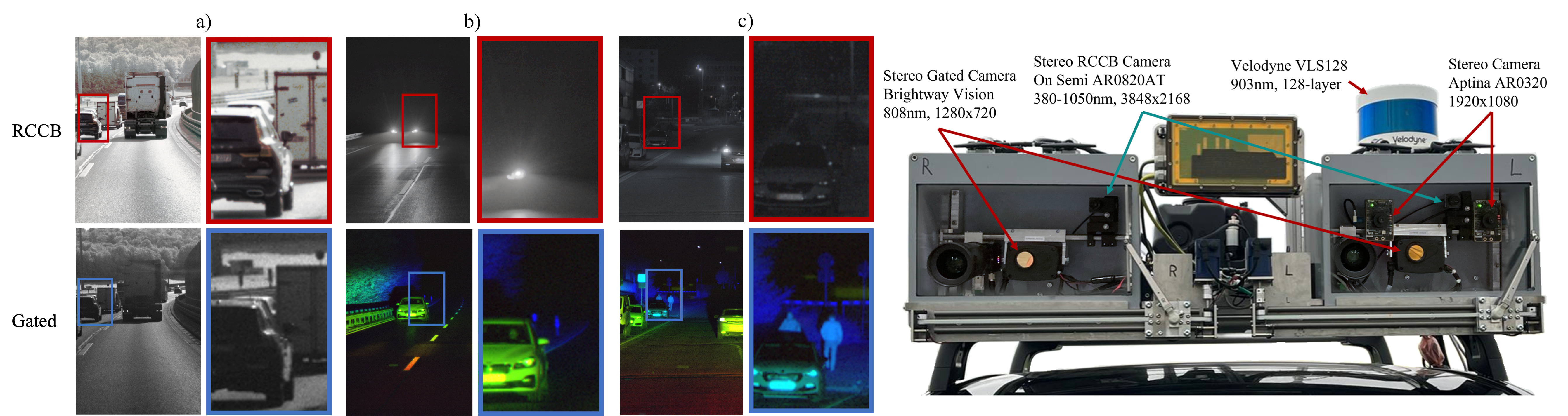
Experimental Results in Diverse Driving Conditions
In the following, qualitative results of the proposed Gated RCCB Stereo are compared to existing methods that use gated images (Gated2Gated, Gated Stereo), stereo RCCB images (CREStereo) as input. Gated2Gated uses three active gated slices and demonstrates effective exploitation of implicit depth cues in gated images. Gated Stereo uses the same gated stereo images as our method, combining gated and multi-view cues. CREStereo (RCCB) is the next best stereo depth estimation method for high-resolution RCCB data as input.
Daytime Suburban Environment
Nighttime Urban Environment
Lost Cargo
Depth estimation for ”lost cargo”, small objects at far distances on ground level that may be lost from preceding vehicles. Displayed below are two scenes demonstrating lost cargo, achieved by positioning small objects at varying distances. The examples depict a tire at 55 meters during the night and a fallen bicycle at 100 meters during the day. Our method estimates accurate depth for these small objects in both daylight and nighttime conditions by integrating complementary RCCB and gated images. Single modality methods suffer from limitations: CREStereo (RCCB) lacks effective illumination at night, and Gated Stereo suffers from poor resolution during the day.
Cross-Spectral Stereo Matching Network
The proposed cross-spectral stereo architecture estimates depth from stereo RCCB and stereo gated images. Intermediate depth estimates are used for iterative fusion within the Cross-Spectral Matching (CSM) layer along the depth estimation process. The network is trained with self-supervision (Left-Right consistency for RCCB and gated images, Gated Reconstruction) and LiDAR supervision.
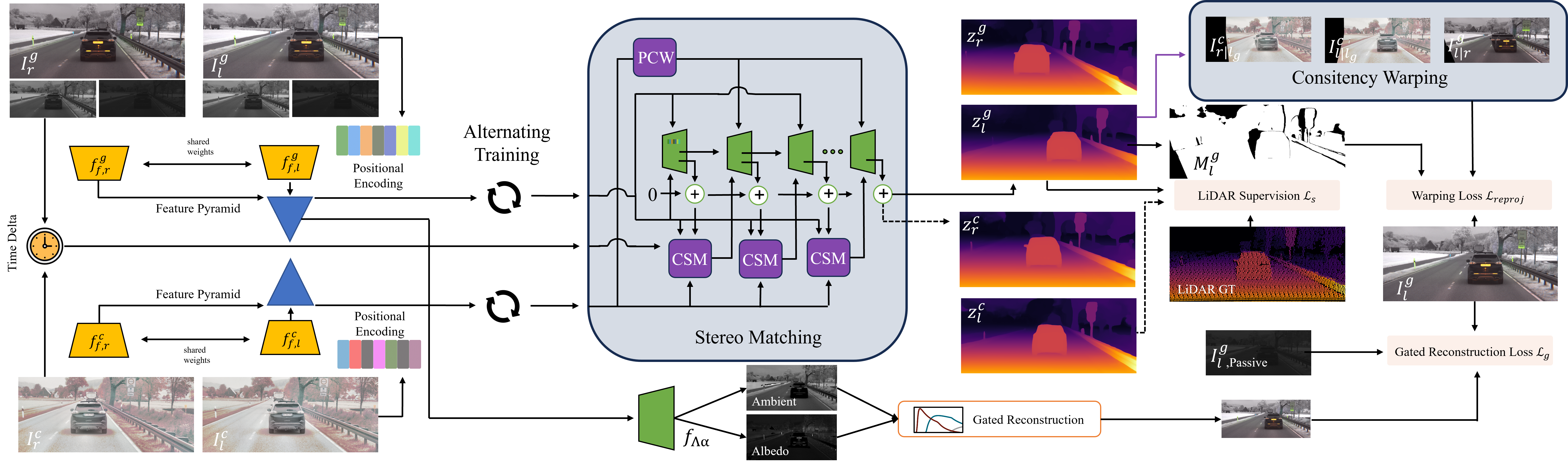
The Cross-Spectral Matching (CSM) layer fuses encoded features from RCCB ($F^c_l$) and gated ($F^g_l$) images. In the coarse registration step, RCCB features are aligned with gated features based on calibrated poses $X_{c \to g}$. Registration is refined based on residual pose $\hat{X}_{c|g \to g}$ estimated from coarse aligned images and measured time delta with PoseNet. Registered images are fused with attention-based fusion retaining complementary information in $\hat{F}$.

Zoom-In Depth Maps
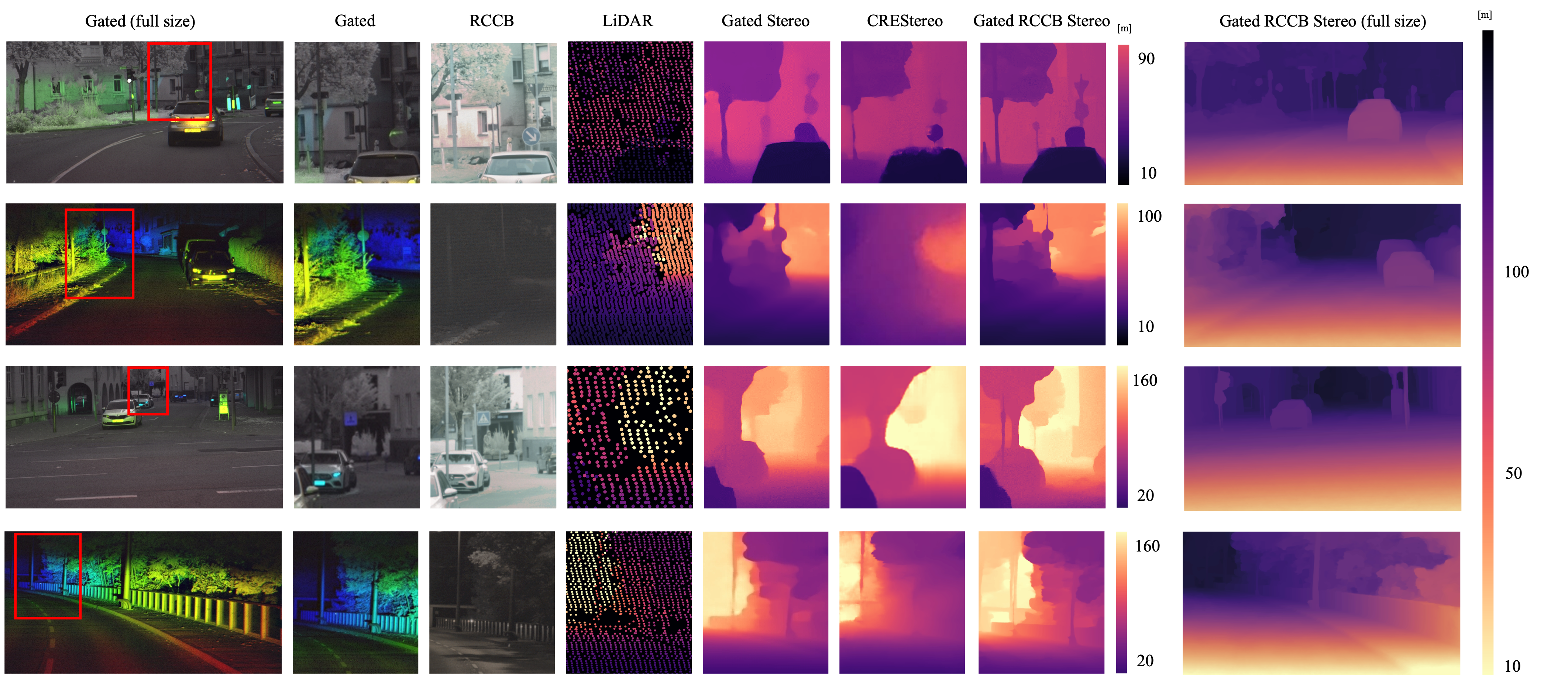
Related Publications
[1] Stefanie Walz, Mario Bijelic, Andrea Ramazzina, Amanpreet Walia, Fahim Mannan and Felix Heide. Gated Stereo: Joint Depth Estimation from Gated and Wide-Baseline Active Stereo Cues. The IEEE International Conference on Computer Vision (CVPR), 2023.
[2] Amanpreet Walia, Stefanie Walz, Mario Bijelic, Fahim Mannan, Frank Julca-Aguilar, Michael Langer, Werner Ritter and Felix Heide. Gated2Gated: Self-Supervised Depth Estimation from Gated Images. The IEEE International Conference on Computer Vision (CVPR), 2022.
[3] Jiankun Li, Peisen Wang, Pengfei Xiong, Tao Cai, Ziwei Yan, Lei Yang, Jiangyu Liu, Haoqiang Fan and Shuaicheng Liu. Practical Stereo Matching via Cascaded Recurrent Network with Adaptive Correlation. The IEEE International Conference on Computer Vision (CVPR), 2022.
[4] Tixiao Shan, Brendan Englot, Drew Meyers, Wei Wang, Carlo Ratti and Daniela Rus. LIO-SAM: Tightly-coupled Lidar Inertial Odometry via Smoothing and Mapping. The IEEE/RSJ International Conference on Intelligent Robots and Systems (IROS), 2020.